ARIN2610 – Internet Transformations
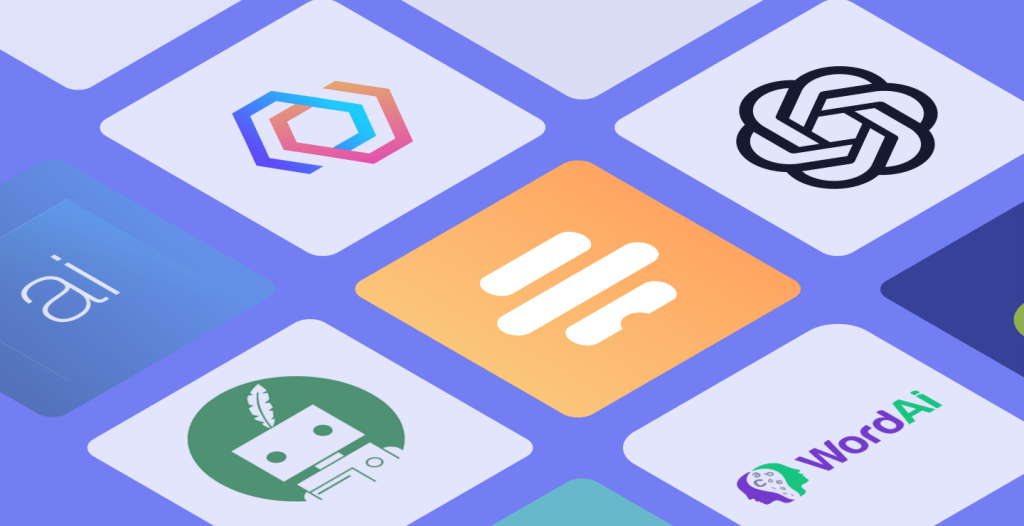
“Artificial Intelligence & AI & Machine Learning” by mikemacmarketing is licensed under CC BY 2.0.
The origin & development of structual biases on Internet images from searching engine
With the continuous evolution of the scale of the Internet, search engines are gradually becoming a new paradigm for people’s online navigation. As the most widely used source of network images and a guide for users, its unique algorithmic logic hides behind it, allowing some biased images to be reflected in its search results through its encoding-decoding-re-encoding feedback mechanism (Heilweil, 2020). Meanwhile, as part of a participatory culture, with the early lack of censorship mechanisms for content release on the Internet, many biased and aggressive images have also been left in the vast information base of the Internet. As shown by Fortunato et al. (2006), this creates a vicious spiral that amplifies the dominant position of existing and popular biased images. At the same time, the expansion of influence over these images has further shaped systematic biases from search engines, which are concentrated on gender, occupational biases, racism, and political positions. According to Schwemmer et al. (2020), a survey of visual representation biases between males and females in image search systems indicates that previously existing stereotypical gender concepts will be used primarily in image push, leading to further structural biases. Although in recent years, Google has begun to adjust its image search results (Grant, 2021), attempting to increase occupational profiling of diverse genders, races, and sexual orientations, such as the image search results of typical STEM scientists (as shown in first image below). However, based on the current search results (as shown in the second image below), structural bias against gender is still evident and continues to dominate the continuation of structural bias.
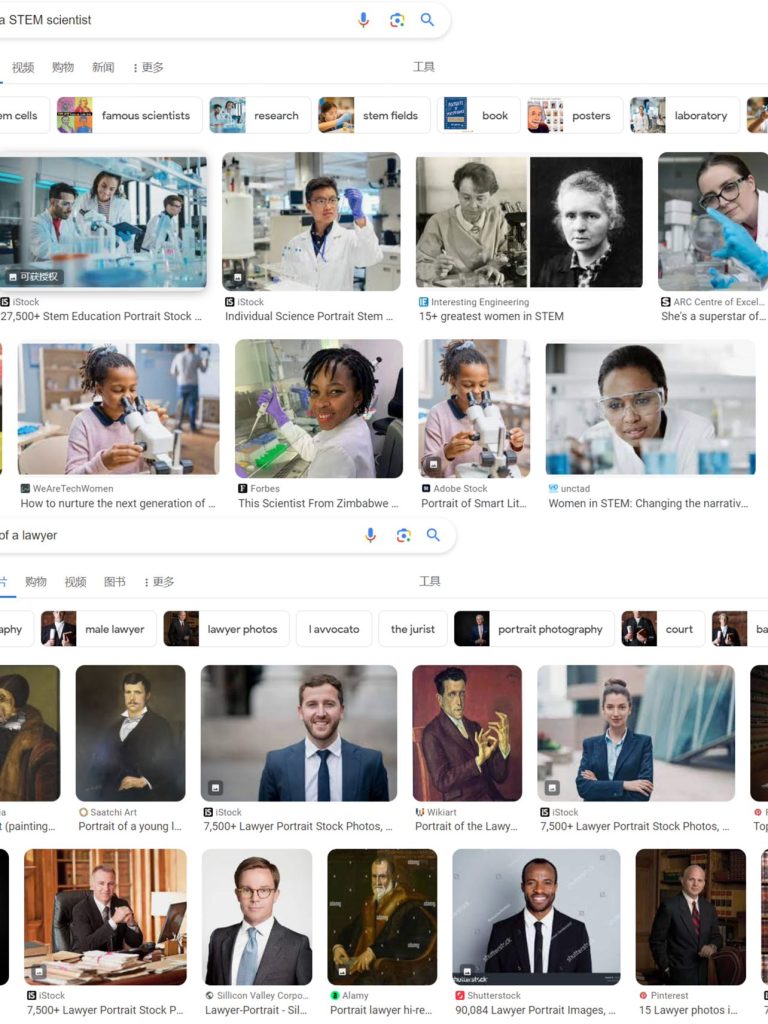
No CC license, direct screenshot of Google’s image search results for searching purpose
The shifting & causes of structural biases: AI image geneators
Similarly, with the rise of AI tools, people have gradually discovered that structural biases in image results are not only reflected in search engines. AI image generators, as an online tool for creating images based on text input, also produce images that have structural biases similar to search engines. The biases of gender and race have been perpetuated here. The reason for this is that due to AI’s self-learning tendency and negative feedback loop, where a content creation cycle is exacerbated by its negative content influence based on biased content feedback and re-coding posts, systematic bias is always rooted in the images created by AI. According to Thomson& Thomas (2023), except for external negative feedback guidance, the lack of diversity in the underlying training dataset also leads to the emergence of structural biases. As the researchers have concluded,
“Most of the time, bias is not intentional. Data represent our world: if they are biased, it is most often because our world itself is biased”
(Devillers et al., 2021)
It is obvious that due to the complex causes of structural biases and the increased influence of AI tools, the influence of negative biases is exacerbated and continues to affect the real world. The following video reflects the complex factors of AI bias and representation for different groups during AI image creation.
Representation of biases from AI image geneators: racialism & gender stereotypes.
When it comes to the specific manifestation of structural bias in AI image generators, it is closely linked to factors such as gender, occupation, race, and nationality. A set of data from Nikolic& Jovicic (2023) accurately displays gender biases related to professions in the generated images. According to its test results, in the career Portrait created by AI Image Generator about STEM scientists, males occupy an absolutely dominant position, which is not consistent with the 28% to 40% proportion of females in reality. As Sun et al. (2023) found, the structural biases embedded in images based on algorithmic recommendations lead to the binding between stereotypes and gender, and through additional facial and gesture representations, shape the potential emotional and status dependencies between different genders, making it more in line with traditional cultural expectations. These all reflect the contribution of AI image generators in addressing structural biases. According to the real-world cases of AI image generators conducted by Growcoot (2023) and Heikkila (2023) based on different occupational profiles in 2023, the vast majority of industries, especially those that are closely related to occupational stereotypes in traditional consciousness, exhibit structural biases in their image generation results. Typical examples include CEOs, professors of different subjects, and when additional adjectives are used as input variables, their gender correlation is particularly prominent. The Twitter posts below also reflect the emergence of this correlation between gender and specific careers very well.
On the other hand, the occupational bias brought by skin color is also evident. According to Nicoletti&Bass (2023), the AI generation test of occupational images based on skin color shows that people with lighter skin colors in occupational profiles of different races represent the theme of high-paying jobs, while those with darker skin colors correspond to jobs with base salary or even criminal occupations, which is not consistent with the actual proportion and situations, Presenting a systematic bias of excessive representation. From the conclusion of Corner& Liu (2023), it can be seen that AI generation systems based on human experience are inevitably dependent on traditional bias and discuss the bias shaping caused by racial data differences. From the current perspective, these main structural biases are currently prevalent in the results of AI image generators, and the use of AI tools has increased to consolidate their own stereotype shaping and influence.
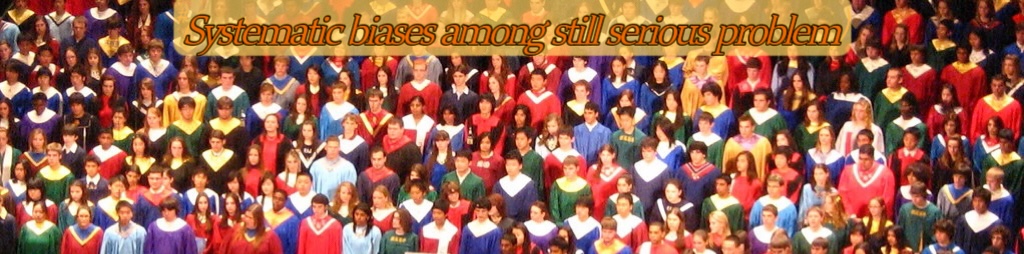
“Colorful people” by *PaysImaginaire* is licensed under CC BY-ND 2.0.
Critical views: Oppotunities & Essential developments for structural baises of AI tools
From a critical perspective, the existing structural biases exhibited by AI image generators have not only caused negative impacts. From the perspective of future development, there is also a necessity for the existence of constitutive bias as the cornerstone of future content creation & censorship. According to Hagendorff &Fabi’s (2022) technical viewpoint,
“When considering complex real-world environments, human biases, and heuristics can be turned from a phenomenon that has to be avoided into a tool that might improve decisions, which is transferable in a certain way to artificial learning systems”
(Hagendorff &Fabi, 2022)
They believe that the feedback mode of creating content’s secondary encoding can reasonably increase AI algorithms’ awareness of different forms of bias and thus accelerate the growth of AI tools. By providing feedback on systemic biases to avoid the continuation of future prejudice creation, which coincides with Johnson’s (2022) view that the production of images that overly conform to cognitive biases should be avoided through learning from diverse datasets and reducing the accuracy of AI image generators. Referring to the algorithm learning improvement of search engines, this makes it necessary for existing systematic biases to exist and take into account future development needs, making constructive contributions.
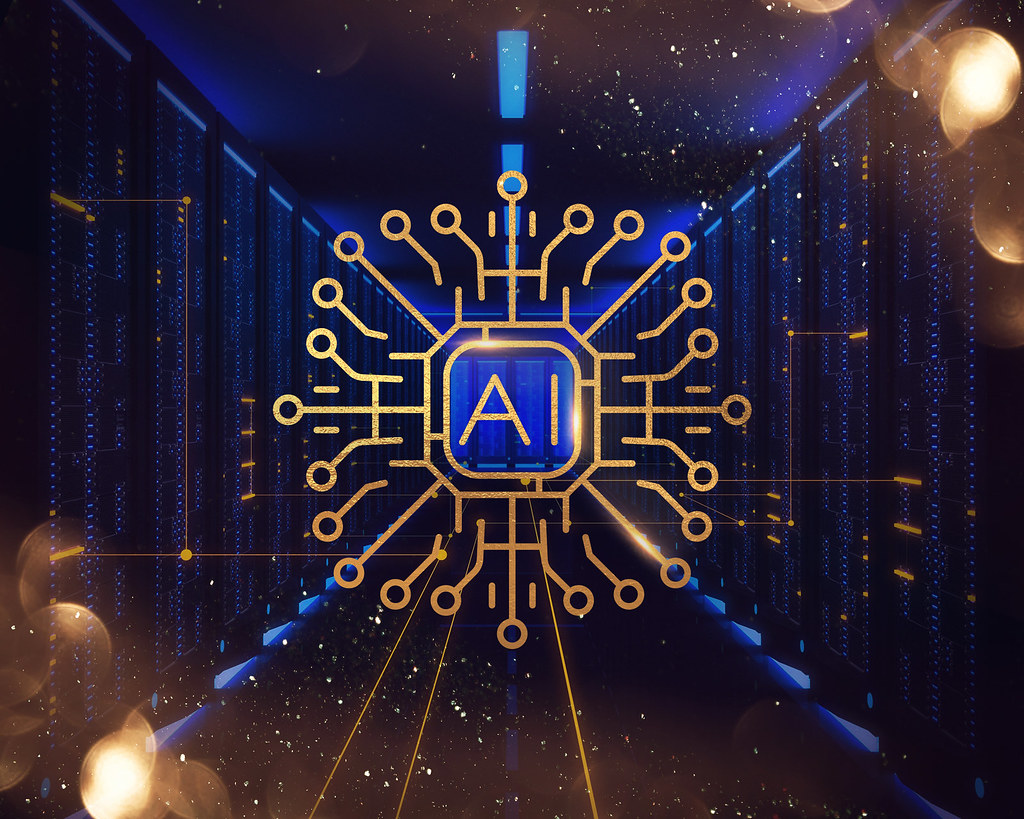
“Artificial Intelligence & AI & Machine Learning” by mikemacmarketing is licensed under CC BY 2.0.
Free discussion: Introduction of Platform Governance and Possible Future Improvement Directions
Although the structural bias of AI image generators seems to be a difficult problem to solve at this stage。 However, the discussions on their future development are still valuable. As an open platform, introducing a monitoring mechanism for image content may be an effective way to address bias. As stated by Cheung (2023), introducing third-party monitoring agencies to handle complaints against biased content to form positive feedback may be a beneficial perspective. In addition, according to Gorwa (2019), introducing multi-party regulation can diversify the development of content review, while also taking into account public trust and the quality requirements imposed on AI training datasets under standardization, which would be a helpful strategy. Meanwhile, as found by Lamensch (2023), the Open AI team, which is currently the largest AI image generator team, is also considered a major cause of algorithmic bias due to the severe lack of diversity and representativeness within. This founding team composed of mostly white males is also considered as a symbol of the lack of representativeness behind the structural bias brought by the underlying algorithms (See image below). Considering Gross’s (2023) suggestion on algorithm bias, the engineering team must realize that algorithms are not completely neutral, and the dataset used should undergo careful planning and consider the bias cycle caused by external access. Overall, AI image generators still have serious structural biases at this stage, and considering their development goals, new mechanisms, and designs need to be introduced in the future to improve the current problems.
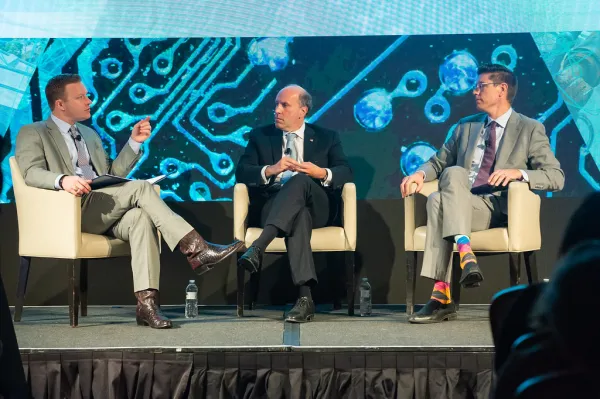
“AI Innovation XLab” by Argonne National Laboratory is licensed under CC BY-NC-SA 2.0.
Reference List
Heilweil, R. (2020, February 18). Why algorithms can be racist and sexist. VOX.
https://www.vox.com/recode/2020/2/18/21121286/algorithms-bias-discrimination-facial-recognition-transparency
Grant, N. (2021, October 20). Google Quietly Tweaks Image Searches for Racially Diverse Results. Bloomberg. https://www.bloomberg.com/news/articles/2021-10-19/google-quietly-tweaks-image-search-for-racially-diverse-results
Fortunato, F., Flammini, A., Menczer, F., & Vespignani, A. (2006). Topical interests and the mitigation of search engine bias. PNAS, 103(34), 126984-12689. https://doi.org/10.1073/pnas.0605525103
Schwemmer, C., Knight, C., Bello-Pardo, D. E., Oklobdzija, S., Schoonvelde, M., & Lockhart, W. J. (2020). Diagnosing Gender Bias in Image Recognition Systems. Sage Journal, 6. https://doi.org/10.1177/2378023120967171
Thomson, T. J., & Thomas, R. J. (2023, July 10). Ageism, sexism, classism and more: 7 examples of bias in AI-generated images. The Conversation. https://theconversation.com/ageism-sexism-classism-and-more-7-examples-of-bias-in-ai-generated-images-208748
Devillers, L., Soulie, F. F., & Baeza-Yates, R. (2021). AI & Human Values. In Braunschweig, B., Ghallab, M. (eds.), Reflections on Artificial Intelligence for Humanity.(pp.76-89). Springer Link. https://doi.org/10.1007/978-3-030-69128-8_6
Nicolic, K., & Jovicic, J. (2023, April 3). Reproducing inequality: How AI image generators show biases against women in STEM. UNDP Serbia. https://www.undp.org/serbia/blog/reproducing-inequality-how-ai-image-generators-show-biases-against-women-stem
Sun, L., Mian, W., Sun, Y., Yoo, J. S., Shen, L., &Yang, S. (2023). Smiling Women Pitching Down: Auditing Representational and Presentational Gender Biases in Image Generative AI. Cornell University Library, arXiv.org. https://www.proquest.com/docview/2815842661 accountid=14757&parentSessionId=SXet4K4ybRQoyXeNacvVoM57tWb16OBPNkaqYnGbsH4%3D&pq-origsite=primo
Growcoot, M. (2023, May 4). AI Reveals its Biases by Generating What it Thinks Professors Look Like. Petapixel. https://petapixel.com/2023/05/04/ai-reveals-its-biases-by-generating-what-it-thinks-professors-look-like/
Heikkila, M. (2023, March 22). These new tools let you see for yourself how biased AI image models are. MIT Technology Review. https://www.technologyreview.com/2023/03/22/1070167/these-news-tool-let-you-see-for-yourself-how-biased-ai-image-models-are/
Nicoletti, L., & Bass, D. (2023, April 25). Humans are biased, Generative AI is even worse. Bloomberg. https://www.bloomberg.com/graphics/2023-generative-ai-bias/
Corner, O. S., & Liu, H. (2023). Gender bias perpetuation and mitigation in AI technologies: challenges and opportunities, AI & Scoiety 23(4). https://doi.org/10.1007/s00146-023-01675-4
Hagendorff, T., & Fabi, S. (2023). Why we need biased AI: How including cognitive biases can enhance AI systems. Journal of Experimental & Theoretical Artificial Intelligence, 1-14. https://doi.org/10.1080/0952813X.2023.2178517
Johnson, K. (2022, May 5). DALL-E 2 Creates Incredible Images—and Biased Ones You Don’t See. Wired.
https://www.wired.com/story/dall-e-2-ai-text-image-bias-social-media/
Lamensch, M. (2023, June 14). Generative AI Tools Are Perpetuating Harmful Gender Stereotypes. Centre for internatioal Goverance Innovation. https://www.cigionline.org/articles/generative-ai-tools-are-perpetuating-harmful-gender-stereotypes/
Cheung, J. (2023, August 21). How AI Image Generators Make Bias Worse. The London Interdisciplinary School. https://www.lis.ac.uk/stories/how-ai-image-generators-make-bias-worse
Gross, N. (2023). What ChatGPT Tells Us about Gender: A Cautionary Tale about Performativity and Gender Biases in AI. Social Sciences, 12(8), 435. https://doi.org/10.3390/socsci12080435
Gorwa, R. (2019). The platform governance triangle: conceptualising the informal regulation of online content. Internet Policy Review, 8(2). DOI: 10.14763/2019.2.1407
Witty Works | Inclusive Writing Assistant [@witty_works]. (2023, August 10). A great research done about bias in imagery from four different AI image generators. [Tweet]. Twitter. https://twitter.com/witty_works/status/1689290439579303937
LIS – The London Interdisciplinary School. (2023, September 5). How AI Image Generators Make Bias Worse. [Video]. YouTube. https://www.youtube.com/watch?v=L2sQRrf1Cd8