Introduction
Within the world today, people turn to Google whenever they need information. The days of flipping through a physical book, being able to hand-select which chapters you look at, and making your own judgments without other commenters are a concept of the past. Though using the internet is far more convenient and efficient than conducting research through books, there are aspects of the internet that cause more harm than good. Research has found that search engines are not foul-proof, and human biases can still exist in software that is supposed to be an impartial actor. Overall, search engines and AI software contain biases that bolster racist ideals, furthering hate online that infiltrates into the physical world (Gray, 2019).
Biases in search engines
Society has deep trust in search engines, with people turning to the softwares for a plethora of different information. Search engines like Google are resources used by the public that promote collaboration, but they are wired to perpetuate harmful stereotypes and bolster racist ideals. Google is an advertising agency with economic obligations to powerholders, leading the software to be biased towards content that promotes whiteness (Gray, 2019). Google can prioritize search engine results that support the principles and success of their economic backers, and “dominant narratives reflect the kinds of hegemonic frameworks and notions that are often resisted by women and people of color” (Noble, 2018). For example, a search done on “Black girls” led to a highly sexualized result, even though the search contained no sexual or pornographic references. Furthermore, Search engines are considered to be fair and impartial, but the reality is that they filter information in a biased way, creating ramifications that lead to continued oppression (Gray, 2019).
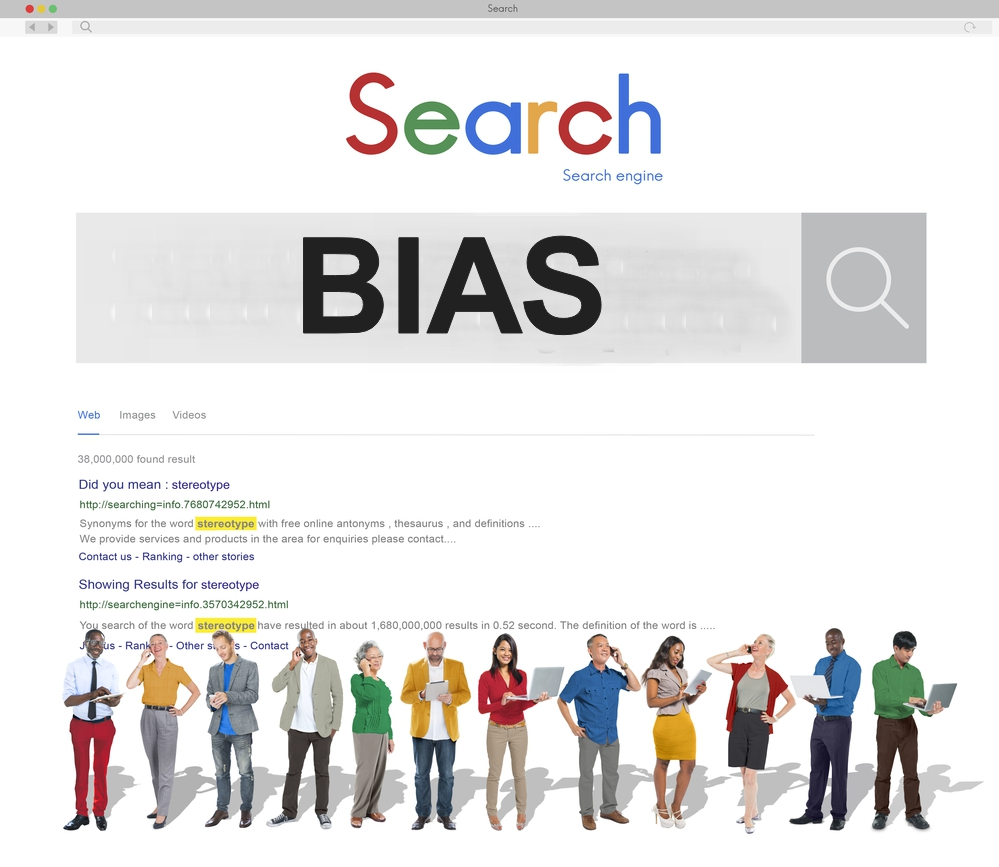
Examples of biases in search engines
The biases that are rooted in search engines such as Google are a direct cause of violence in the physical world. In a review done on search engines by the information company Alexa, the first three page results of the searches “Holocaust,” “Islam,” “White people,” and “Black people” were examined within the search engines Google, Yahoo, Bing, and Ask.com. The study found that each search result contained at least two websites that were funded by white supremacy groups or racist groups (Klein, 2012). By promoting racist ideas on the internet, search engines are, even if inadvertently, fueling racism in the physical world. Building on this idea, an apparent example and evidence of the danger of biased search engines is the Dylann Roof case, in which a young man named Dylann Roof killed nine black individuals at his church. Roof credited his ability to carry-out a terrorist attack to his accessibility to websites, and he was influenced and encouraged by white nationalistic websites (Kara, 2019). Furthermore, “there is evidence that white nationalist sites are willing to pay Google more, and/or put more time into SEO, than the FBI” (Kara, 2019), exhibiting that Google is willing to bolster the power of racist sites for an economic gain. It is clear that search engines perpetuate racism, and the consequences are dire.
Biases in AI technologies
Building on biases in search engines, AI technologies that are designed to be “unbiased” have been found to contain racist tendencies, exposing that technological biases are contributing to prejudices in the physical world (Levin, 2016). For example, an international beauty competition made up of people from over 100 countries was set to be judged by robots in order to prevent racial biases within beauty. However, the results displayed massive biases, with almost all 44 of the winners being white and only one having dark skin (Levin, 2016), even though the algorithm allegedly did not evaluate skincare (Pearson, 2016). The results of this beauty contest display that softwares meant to be impartial can still be full of human biases.
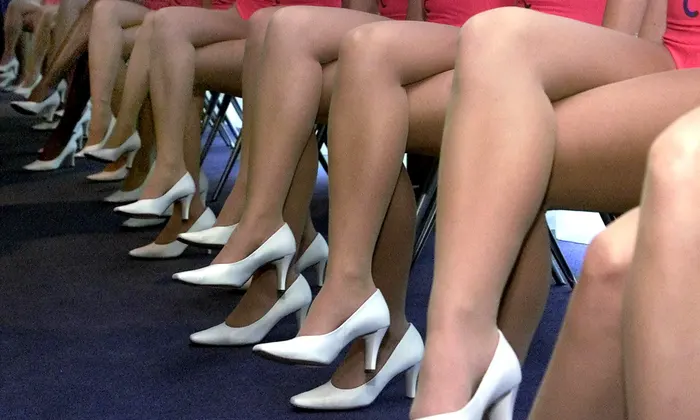
The benefits of biases in search engines
Though search engine biases are highly detrimental, there is evidence that biases in the software are necessary and beneficial (Goldman, 2006). In order for search engines to work and deliver the most effective result, the software will “tune their ranking algorithms to support majority interests” (Goldman, 2006). It’s very difficult for search engines to filter through every result on the internet, so using popularity metrics to gauge what is popular is a more efficient way to deliver the most popular result. Furthermore, without biases in search engines, the internet could be quickly taken over by hacking and spam, which would cause unrest and disorder. Thus, biases in search engines allow the internet to distribute third party content in a way that is beneficial to users. It is crucial for search engines to be biased toward popular websites because they are more likely to be of interest to the greatest number of users (Goldman, 2006).
Personalization of search engines
Though popularity metrics create structure and organization within search engines, this causes “results to be biased towards websites with economic power” (Goldman, 2006), which boosts the power of already large websites, while dwindling the influence of smaller sites (Goldman, 2006). Thus, a possible approach to combat search engine bias is to create personalized algorithms because it lessens the ability to create website “winners” and website “losers” (Goldman, 2006). With personalized search engines, people looking for information from less popular websites will be able to find it, and search results will reflect “heterogeneous search objectives”’ (Goldman, 2006).
Personalized search engines are a step forward in combating harmful search engine bias, yet they are not a full-proof approach. Even though search engines are personalized, people still have implicit bias which leads to “sustaining and supporting our conditioning for the maintenance of white masculine supremacy” (Gray, 2019). Building on this idea, personalized search engines introduce the problem of confirmation bias, and it leads to “excessive personalization leads to never seeing the other side of an argument and thus fostering an ill-informed political discourse” (Bozdag, 2013). Therefore, though personalized search engines can help avoid filtering certain websites to the top and weakening smaller websites, it can also be even more detrimental to society by overly-affirming people’s own view and hindering accessibility to different opinions (Bozdag, 2013).
Conclusion
In conclusion, there is clear research exhibiting that search engines and many AI tools are not impartial forms of software, which leads to unrest within the physical world. Though search engines are used by the public, the software companies rely on advertising to profit, which funnels websites with more economic value up in search results, while pushing out smaller sites (Gray, 2019). Furthermore, though personalized algorithms in search engines may offer a glimpse into a more equitable future, biases will still exist. Personalized algorithms run the risk of compounding the issue of confirmation bias and creating a larger divide between people with differing views. Overall, “micronooses, microaggressions, implicit/unconscious bias, covert, and overt oppression are essentially new racisms and stereotypes that have been digitally repackaged and normalized” (Gray, 2019). Until confronted, detrimental power dynamics that perpetuate racism that exist in society will continue to show up in how people search online and what type of results they get, continuing the feedback loop and perpetuating racism online and in the physical world. Different approaches have been brought up to confront the issue of search engine bias, including forcing search engine companies to be more transparent, to publicly-fund search engines, and mandating changes to how websites are ranked (Goldman, 2006). By implementing policies and changing the way that search engines function, the internet will be a more impartial and equitable place to receive information.
References:
Bozdag, E. (2013). Bias in algorithmic filtering and personalization. Ethics and Information Technology, 15(3), 209–227. https://doi.org/10.1007/s10676-013-9321-6
Goldman, E. (n.d.). SEARCH ENGINE BIAS AND THE DEMISE OF SEARCH ENGINE UTOPIANISM. https://yjolt.org/sites/default/files/goldman-8-yjolt-188.pdf
Gray, K. L. (2019). Algorithms of oppression: how search engines reinforce racism. Feminist Media Studies, 19(2), 308–310. https://doi.org/10.1080/14680777.2019.1579984
Jelani Cobb. (2019). Inside the Trial of Dylann Roof. The New Yorker. https://www.newyorker.com/magazine/2017/02/06/inside-the-trial-of-dylann-roof
Klein, A. (2012). Slipping Racism into the Mainstream: A Theory of Information Laundering. Communication Theory, 22(4), 427–448. https://doi.org/10.1111/j.1468-2885.2012.01415.x
Levin, S. (2016, September 8). A beauty contest was judged by AI and the robots didn’t like dark skin. The Guardian. https://www.theguardian.com/technology/2016/sep/08/artificial-intelligence-beauty-contest-doesnt-like-black-people
Noble, S. (2018, March 26). Google Has a Striking History of Bias Against Black Girls. Time; Time. https://time.com/5209144/google-search-engine-algorithm-bias-racism/
Sachdev, N. (2019, November 21). NIST To Measure Bias in Results We Get From Search Engines: “Fair Ranking.” The Sociable. https://sociable.co/web/nist-research-effort-to-measure-bias-in-results-we-get-from-search-engines-fair-ranking/
Alexa.com. (2016). Alexa.com. https://www.alexa.com/
Book Review | Algorithms of Oppression: How Search Engines Reinforce Racism by Safiya Umoja Noble. (n.d.). Retrieved October 7, 2023, from http://eprints.lse.ac.uk/108135/1/dit_com_2019_06_29_book_review_algorithms_of_oppression_how_search.pdf
bloomreach.com. (n.d.). The Importance of Personalized Search. Bloomreach. Retrieved October 7, 2023, from https://www.bloomreach.com/en/blog/2018/the-importance-personalized-search
Why An AI-Judged Beauty Contest Picked Nearly All White Winners. (n.d.). Www.vice.com. https://www.vice.com/en/article/78k7de/why-an-ai-judged-beauty-contest-picked-nearly-all-white-winners