As people get better and better at using code, both in the courtroom and on social media, the principles of programme design are becoming more and more important.
Introduction
The field of internet history has produced a complex body of research with a global reach over the past two decades. A broader understanding of what the web is and the decisions and contingencies that shape it has emerged from empirical studies in different national and regional contexts (ABBATE, 2017). AI Artificial intelligence is growing in popularity under the boom of networks, and artificial intelligence (AI) is profoundly transforming industries and reshaping the way we live our daily lives. While AI has the potential to bring about positive change, it is not immune to the social biases and prejudices that exist in the real world. For example, AI algorithms run the risk of reinforcing social biases (Zou and Schiebinger, 2018).
AI algorithms learn patterns and make predictions based on training data, including machine learning models and deep learning networks. The AI system inadvertently perpetuates or even exacerbates these biases if the training data reflects historical racial biases. The world’s first international beauty pageant judged by AI was born out of such an original idea. But a number of controversies have also arisen from the AI’ decisions.
Prejudice triggered by beauty contests
Sometimes biases are hard for people to see, but sometimes they show. A beauty contest called Beauty.ai by Youth Laboratory attracted 600,000 entries under the banner of artificial intelligence judging.Sponsored by tech giants NVIDIA and Google among others, the contest was judged by robots called Beauty AI.Contestants had to download the Beauty AI app, take a selfie, and Beauty. AI 2.0 evaluated 6,000 selfies from more than 1,000 countries, using algorithms that took into account age, skin tone, symmetry and other factors. It also compared the contestants’ appearance to that of actors and models, and ultimately grouped the winners by age and gender. However, ethnicity appeared to be a bigger factor than expected when it was found that the robots had a bias in favour of contestants with lighter skin. 36 out of the 44 winners were white. This bias was attributed to the deep learning algorithms that were used to judge the photos, which had been obtained by training on images that were pre-tagged.
Sometimes bias is hard for people to see, but sometimes it shows. A beauty contest called Beauty.ai , launched by Youth Laboratory, attracted 600,000 participants under the banner of artificial intelligence judging.Sponsored by tech giants NVIDIA and Google , among others, the contest was judged by robots called Beauty AI.Participants had to download the Beauty AI app, take a selfie, and have their photo judged by a Beauty. AI 2.0.The robot scored 6,000 selfies from over 1,000 countries, using algorithms that looked at age, skin tone, symmetry and more.It also compared people’s looks to actors and models, and finally grouped winners by age and gender. But ethnicity appeared to play a bigger role than expected when it was discovered that the robots preferred light-skinned candidates. 36 out of the 44 winners were white. The deep learning algorithms used to judge the photos, which were trained on images with pre-selected colours, were blamed for this bias.
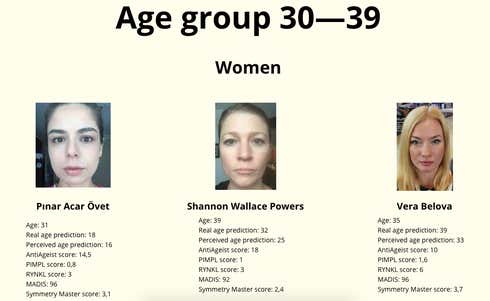
The results of the beauty.ai competition. is licensed under CC BY-NC-ND 4.0.
Motherboard describes the AI’s algorithms for judging “beauty” as using a type of machine learning called deep learning. In deep learning, an algorithm is ‘trained’ on a set of pre-labelled images. This means that when it is presented with a new image, it can be reasonably sure of what it is seeing. In the case of Beauty.ai, all of the algorithms were trained on an open source machine learning database that was shared between the researchers, and the AI revealed a number of problems. Firstly, the documentation mentions the problem of racial bias in AI, when robots show a preference for light-skinned contestants in robotically judged beauty pageants (benjamin, 2023). This is because the deep learning algorithms used to judge the photos are trained on labelled images (benjamin, 2023). As a result, the robot’s judgement is influenced by social biases, and these biases relate not only to attractiveness, but also to areas such as health, intelligence, crime and employment (benjamin, 2023). the study found that in the facial recognition algorithms, the AI showed biases similar to the Other Race Effects (ores) that have been observed in humans. the ores refer to the fact that people are more attracted to and better able to recognise the faces of their own race than faces of other races (tian, 2021). The researchers showed that the AI was better at recognising white faces and worse at recognising black and Asian faces when trained on a dataset containing more white faces. However, when the dataset was balanced or contained more Asian faces, this bias was reversed and the network performed better at Asian face recognition (Tian, 2021).
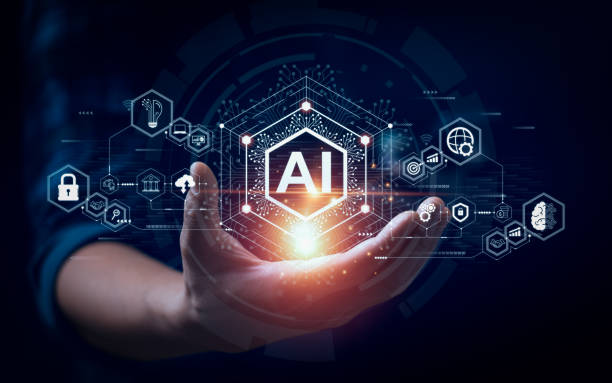
Shutthiphong Chandaeng by Getty Images/iStockphoto is licensed under CC BY-NC-ND 4.0.
How Artificial Intelligence Bias Reflects Social Bias
‘’If a system is learning based on a majority of white photos, it is bound to struggle to identify black photos,” AI systems can make biased predictions based on AI systems can make biased predictions based on race, which reinforces existing biases and perpetuates harmful stereotypes.AI is not just biased in terms of looks, but also in the areas of health, intelligence, crime and employment (Benjamin, 2023). For example, only 11 per cent of people who appear in Google image searches for the term ‘CEO’ are women. A few months later, Anupam Datta conducted an independent study at Carnegie Mellon University in Pittsburgh and found that Google’s online advertising system was much more likely to show high-paying jobs to men than to women, with Apple credit card problems and DeepDream experiment. Friedman and Nissenbaum (1996) showed that software can systematically and unfairly discriminate against some individuals or groups in favour of others. Bias can manifest itself in computing systems in a number of ways. Pre-existing societal biases may influence system design, technological biases may arise because of technological constraints, and emergent biases may arise at some point after the software implementation is completed and published (Friedman and Nissenbaum 1996). The causes of bias in AI can be attributed to data bias and algorithmic bias. Data bias is a bias or imbalance in the training data used to train machine learning models. This can be caused by sample selection bias when collecting data, incomplete or inaccurate data, or the dataset itself reflecting unfair societal or systemic biases. (Loza, 2021).Algorithmic bias is when a machine learning system makes a prediction or decision that has the potential to have an unfair or discriminatory impact, based on the patterns and associations in the training data. This can be caused by the model focusing too much on certain groups or specific characteristics, or by biases in the training data. On the other hand, data bias and algorithmic bias are the main factors that can lead to bias in AI, which can have an unfair impact on the decision-making system (Loza, 2021).
In conclusion
Addressing social bias and racism in artificial intelligence (AI) systems requires a number of strategies. First, recognition and awareness of the existence and consequences of racial bias in AI systems is crucial. Highlighting cases such as robot-judged beauty contests that favour lighter skin can help shed light on this issue. RETHINKING THE RELATIONSHIP BETWEEN TECHNOLOGY AND RACE: We need to recognise that technology is not neutral and that it can both reflect and perpetuate social prejudices. Addressing racial bias in AI will require a deeper understanding of and research into the ways in which race is embedded in the design and development of technology ((Benjamin, 2023). Technically, we need to balance training datasets to ensure they include facial images from multiple races, and try to keep the number of images from different races as close together as possible. We can also use new algorithms to tune the internal representation of the DCNN to reduce bias. (Tian, 2021)). Finally, human-selected data or data with social or historical differences can be used to train models. However, while humans and artificial algorithms validate the data to detect and correct bias, humans are the ones who generate the biased data. However, we can combat AI bias by testing data and algorithms, and by using best practices for data collection, data use, and AI algorithm creation.
Bibliography
References
Benjamin, R. (2019). Race after technology: Abolitionist Tools for the New Jim Code. Polity.
Friedman, B., & Nissenbaum, H. (1996). Bias in computer systems. ACM Transactions on Information Systems, 14(3), 330–347. https://doi.org/10.1145/230538.230561
Hamilton, I. A. (n.d.). Apple cofounder Steve Wozniak says Apple Card offered his wife a lower credit limit. Business Insider. Retrieved October 7, 2023, from https://www.businessinsider.com/apple-card-sexism-steve-wozniak-2019-11?IR=T
Larkin, Z. (2022a, November 16). AI bias – what is it and how to avoid it? Levity.ai. https://levity.ai/blog/ai-bias-how-to-avoid
Larkin, Z. (2022b, November 16). AI bias – what is it and how to avoid it? Levity.ai. https://levity.ai/blog/ai-bias-how-to-avoid
Pearson, J. (2016, September 5). Why An AI-Judged Beauty Contest Picked Nearly All White Winners. Vice. http://motherboard.vice.com/read/why-an-ai-judged-beauty-contest-picked-nearly-all-white-winners
Silberg, J., & Manyika, J. (2019, June 6). Tackling bias in artificial intelligence (and in humans). McKinsey & Company. https://www.mckinsey.com/featured-insights/artificial-intelligence/tackling-bias-in-artificial-intelligence-and-in-humans
Tian, J., Xie, H., Hu, S., & Liu, J. (2021). Multidimensional Face Representation in a Deep Convolutional Neural Network Reveals the Mechanism Underlying AI Racism. Frontiers in Computational Neuroscience, 15(20), 620281. https://doi.org/10.3389/fncom.2021.620281