Sharing Economy: An Introduction
The rapid development of the Internet and the widespread use of artificial intelligence and big data services have given rise to new business models such as the sharing economy. People are entering an economy in which work is broken down into tasks that people around the world perform in their spare time through on-demand service platforms, delivered by freelancers working on multiple platforms or versatile individuals specializing in some other fields (Sundararajan, 2016). The sharing economy is defined as a scalable economy system that provides users with temporary access to intangible and tangible resources through platforms (Eckhardt et al., 2019). The sharing economy has five key characteristics, including providing users with temporary access to resources, transactional activities to make a profit, platforms as intermediaries linking consumers and resource providers, a shift in the identity of users, and a crowdsourcing model in which the supply of resources originates from multiple users (Eckhardt et al., 2019).
The sharing economy is inherently endowed with many positive attributes for its embodiment of values of sharing, decentralization, and green. However, a growing body of research focuses on the negative impacts and outcomes of the sharing economy on social equality and justice. With the rising demand for resource matching and control in sharing economy platforms, AI-led algorithmic management has gradually become the core of these platforms to perform management functions and play an increasingly important role in sharing activities, such as collecting consumer/digital worker/business information, evaluating user preferences, and acting as a proxy for consumer-digital worker activities (Puntoni et al., 2021).
This essay begins with a brief introduction to the sharing economy and the AI-led digital platforms it relies on. Then, this essay continues to discuss how the sharing economy shapes a new form of labor relations and hence creates new issues on labor rights and protection.
Algorithmic Management and Labor Control
With the development of digital technology, the way enterprises control labor has gradually changed to a control method based on platform algorithms, which has become a critical tool for controlling digital labor. Research on crowdsourcing platforms found that the algorithmic management of sharing economy platforms not only diluted employer responsibility and shifted labor conflicts, but also reshaped the labor order through algorithmic learning, weakening the autonomy of digital laborers (Scholz, 2017).
According to existing research, there are mainly three types of factors that contribute to the algorithmic control of digital labor. The first is the compulsory nature of the algorithm. Food-delivery and ride-hailing platforms use algorithms to allocate tasks and plan routes, meanwhile monitoring crowdsourced delivery workers and drivers in real-time. Under the control of algorithms, the delivery workers from Eleme (a major Chinese food delivery platform) only have a formal “right of refusal” to choose whether or not to take on tasks (Li et al., 2020).
The second factor is the opacity of the algorithm. Alex et al. found that delivery workers on the Deliveroo platform were unaware of the process and rationale for task-assigning, and the platform did not explain (Veen et al., 2020). The algorithmic process functions as a black box and brings about irregular tasks that keep digital workers alternating between busy and idle, making them feel anxious and even fearful at times (Scholz, 2017).
The third factor is the induced nature of algorithms. The algorithms of sharing platforms intentionally send additional demand information to digital workers, forcing them to change work schedules and extend working hours. For example, Uber drivers will receive demand information about a certain area at a specific time, stimulating drivers to perform more work (Woodcock et al., 2018).
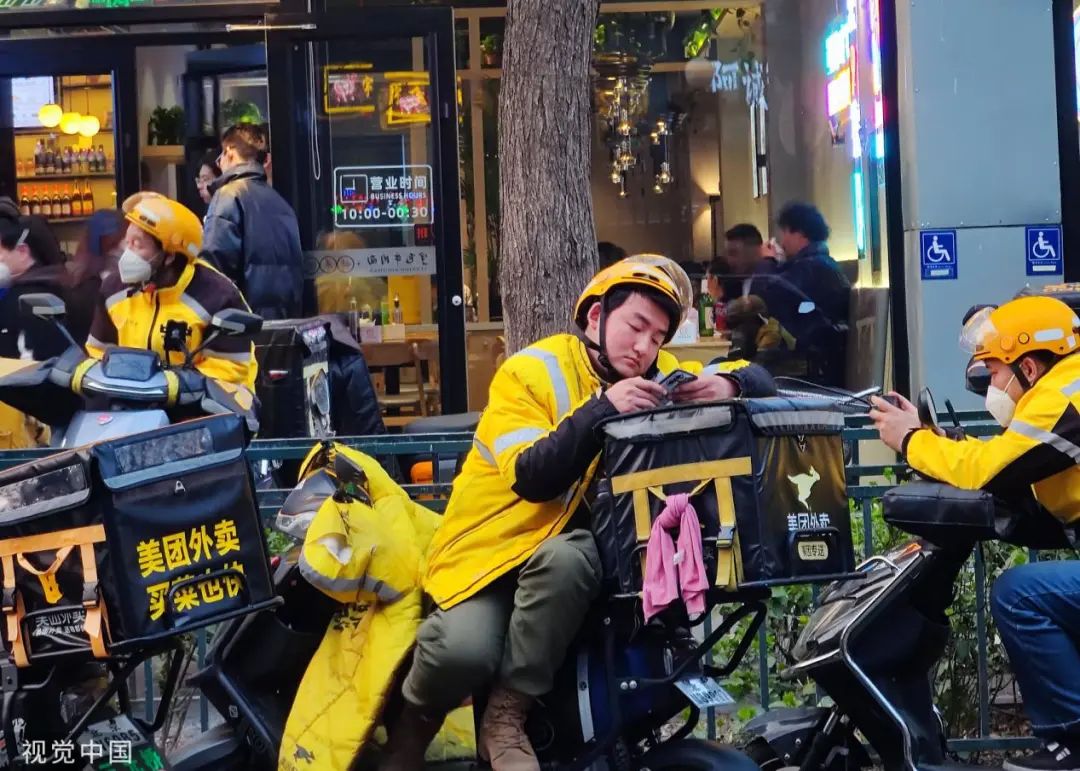
(Food delivery workers in China)
Algorithm Management and Work Performance
The algorithm management of the sharing platform also involves the proxies for performance appraisals. The sharing platforms will use a digital evaluation and reputation system to proxy the performance appraisal of digital works to the consumers.
The inclusion of consumer ratings in the appraisal metrics is closely linked to the performance results and promotions of digital workers. Digital workers can be motivated by consumer appraisals and have the opportunity to improve their consumer ratings through their efforts (Duggan et al., 2020). However, algorithmic performance appraisals also lead to non-objective and unfair appraisal results, which, again, transforms labor conflicts into consumer-worker conflicts.
The consumer-worker conflicts conceal the role played by the algorithm and the platforms in allocating services and resources. Firstly, algorithm-led appraisal systems lack interpretability. Digital workers do not understand how algorithms produce performance results and often question if the results truly reflect their performance (Duggan et al., 2020). Secondly, consumer ratings and appraisals often lack objectivity. The values of consumer appraisal have been exaggerated because the ratings do not provide a realistic picture of the effectiveness of digital laborers’ work. Thirdly, the incentives of platform algorithms lack humanity. As the task volume and rating often contribute to the salary, the pursuit of bigger task volume and higher ratings encourages workers to work overtime with extra costs (Scholz, 2017).
There are researchers point out that the involvement of consumers and vendors creates a complex interaction between consumers, vendors, digital workers, and sharing economy platforms. Throughout the interaction, the platforms reinforce the power of consumers and vendors, making it difficult for digital workers to interact with other parties on an equal footing. Kuhn et al. (2017) argue that digital workers are at the bottom of the power hierarchy and it is difficult for them to gain respect from the other three parties. The rating systems on sharing economy platforms allow consumers and vendors to affect digital workers’ performance through evaluations and complaints. Whereas, digital workers have few channels of feedback, and can only passively accept negative evaluations and reduced pay (Schaefers et al., 2015).
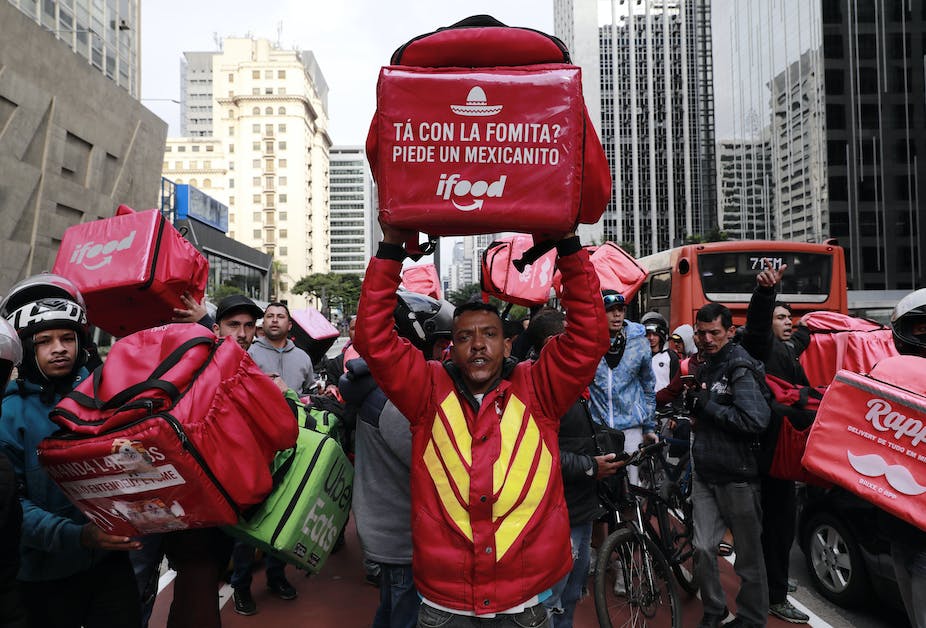
Algorithmic Management and Self-respect of Digital Workers
It is gradually recognized that the algorithmic management of digital workers by the sharing economy platforms can cause negative psychological responses from digital workers.
On the one hand, the sharing economy platform enormously undermines the self-autonomy of the workers. The self-efficacy of employees significantly depends on the autonomy and frequency of participation, and employees with high levels of autonomy tend to have higher levels of efficacy. Under algorithmic management, digital workers can feel the constant surveillance from the platforms and are forced to accept the tasks assigned by the platform algorithm, which reduces their self-efficacy (Möhlmann et al., 2020). Meanwhile, the involvement of consumer and/or vendor’s appraisal embodies a redistribution of control. The power of surveillance and control over digital workers, such as drivers and delivery workers, has been extended from the platforms to consumers and vendors. This extension of surveillance creates a strict scrutiny of work performance and further undermines the autonomy of digital workers (Li et al., 2020).
On the other hand, the sharing economy platform exerts negative influences on the workers’ sense of achievement and justice. Based on the distributive justice perspective, digital laborers would compare their inputs with their returns and expect a fair outcome from the platforms. However, the introduction of algorithmic management in the distribution makes the workers feel underpaid for the effort they put in. Uber drivers, for example, often complain that they receive unfair performance appraisal results and are underpaid (Rosenblat et al., 2016). The complaints derive from the opacity of the appraisal algorithm of the platforms to the digital workers. For instance, Uber uses algorithmic systems to monitor drivers’ speed and location, track their movements and behavior during service, and combine the behavioral data with customer ratings to determine employee pay and performance. However, most drivers are unaware of the fact that their behaviors are monitored to determine their assignment and pay (Lee et al., 2015).
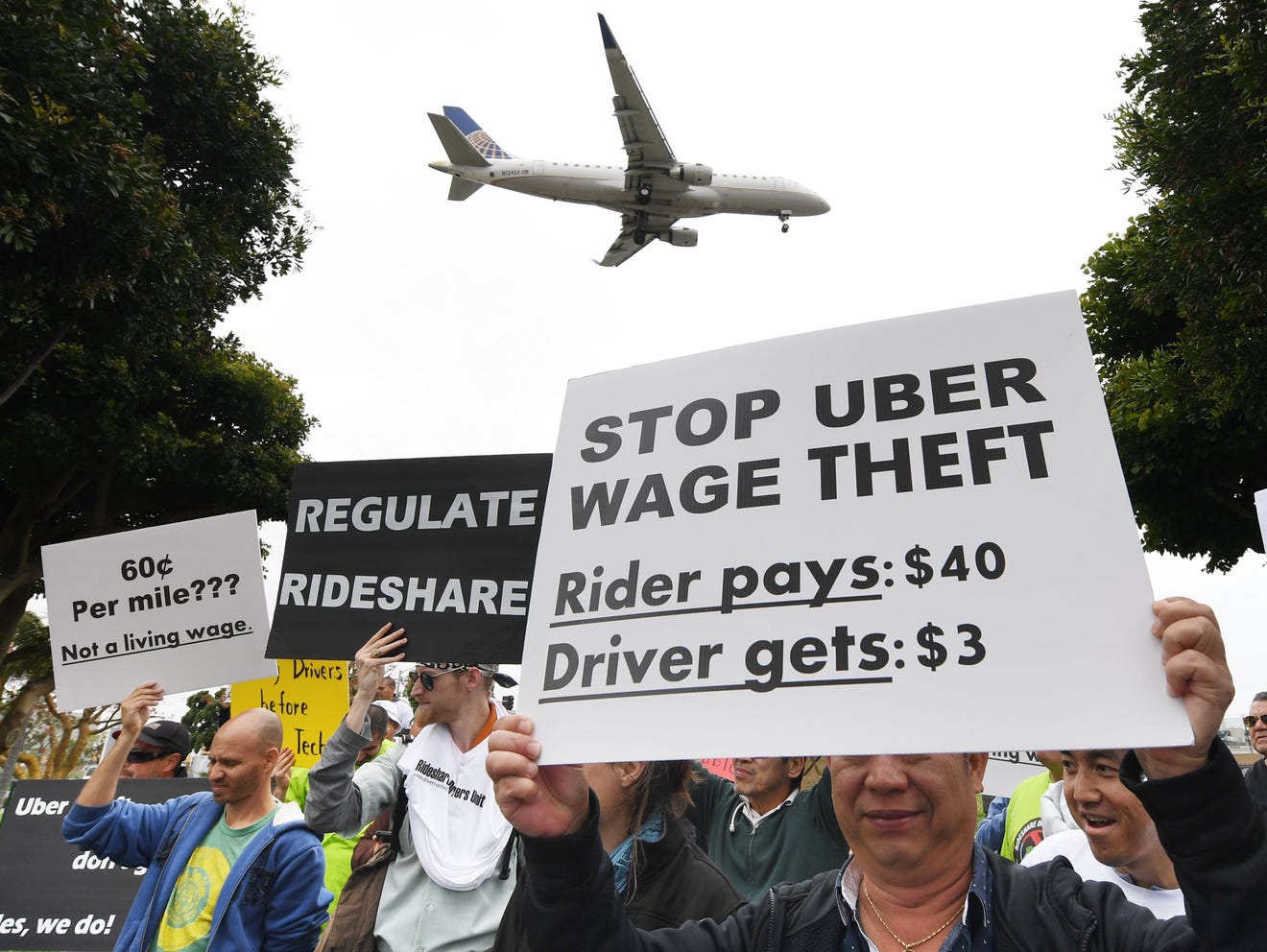
Conclusion
The algorithm-led sharing economy platforms shape a new form of digital labor relations via its unique labor management. These new digital labor relations reflect the negative attributes of the sharing economy in terms of labor rights protection. The algorithmic principle of on-demand work makes it difficult to safeguard the rights and interests of digital laborers in sharing economy platforms. Moreover, the appraisal systems put digital workers in a relatively inferior position to other parties, undermining digital workers’ sense of autonomy and self-efficacy, and exacerbating the power imbalance between digital workers and other stakeholders.
References
Duggan, J., Sherman, U., Carbery, R., & McDonnell, A. (2020). Algorithmic management and app‐work in the gig economy: A research agenda for employment relations and HRM. Human Resource Management Journal, 30(1), 114-132.
Eckhardt, G. M., Houston, M. B., Jiang, B., Lamberton, C., Rindfleisch, A., & Zervas, G. (2019). Marketing in the sharing economy. Journal of Marketing, 83(5), 5-27.
Kuhn, K. M., & Maleki, A. (2017). Micro-entrepreneurs, dependent contractors, and instaserfs: Understanding online labor platform workforces. Academy of Management Perspectives, 31(3), 183-200.
Lee, M. K., Kusbit, D., Metsky, E., & Dabbish, L. (2015, April). Working with machines: The impact of algorithmic and data-driven management on human workers. In Proceedings of the 33rd annual ACM conference on human factors in computing systems (pp. 1603-1612).
Li, C., Mirosa, M., & Bremer, P. (2020). Review of online food delivery platforms and their impacts on sustainability. Sustainability, 12(14), 5528.
Möhlmann, M., Zalmanson, L., Henfridsson, O., & Gregory, R. W. (2021). ALGORITHMIC MANAGEMENT OF WORK ON ONLINE LABOR PLATFORMS: WHEN MATCHING MEETS CONTROL. MIS quarterly, 45(4).
Puntoni, S., Reczek, R. W., Giesler, M., & Botti, S. (2021). Consumers and artificial intelligence: An experiential perspective. Journal of Marketing, 85(1), 131-151.
Rosenblat, A., & Stark, L. (2016). Algorithmic labor and information asymmetries: A case study of Uber’s drivers. International journal of communication, 10, 27.
Schaefers, T., Wittkowski, K., Benoit, S., & Ferraro, R. (2016). Contagious effects of customer misbehavior in access-based services. Journal of Service Research, 19(1), 3-21.
Scholz, T. (2017). Uberworked and underpaid: How workers are disrupting the digital economy. John Wiley & Sons.
Sundararajan, A. (2017). The sharing economy: The end of employment and the rise of crowd-based capitalism. MIT press.
Veen, A., Barratt, T., & Goods, C. (2020). Platform-capital’s ‘app-etite’for control: A labour process analysis of food-delivery work in Australia. Work, Employment and Society, 34(3), 388-406.
Woodcock, J., & Johnson, M. R. (2018). Gamification: What it is, and how to fight it. The Sociological Review, 66(3), 542-558.
Helpful links
Dawson, H.J. (2022). Digital labour platforms subject global South workers to ‘algorithmic insecurity’. The Conversation. Retrieved from
Mix, M. (2019). Column: On Labor Day, consider the injustice of forced union dues. Desert Sun. Retrieved from https://www.desertsun.com/story/opinion/2019/09/01/column-labor-day-consider-injustice-forced-union-dues-mark-mix/2156694001/
New Weekly. (2023). It’s getting harder and harder to make over $10,000 a month delivering takeaways. Retrieved from https://m.thepaper.cn/newsDetail_forward_22505874
Spilda, F.U., Cole, M., Gramham, M., Bertolini, A., Cant, C., Arubayi, D., Salem, N., Reneses, P.A., Warin, R., & Gidda, N. (2022). Most digital labour platforms failing to provide minimum protections for gig workers, finds new Oxford report. Oxford Internet Institute. Retrieved from https://www.oii.ox.ac.uk/news-events/news/most-digital-labour-platforms-failing-to-provide-minimum-protections-for-gig-workers-finds-new-oxford-report/
YouTube. (n.d.). Regulating the digital in labour: Platform work and Algorithm. Socialists and Democrates. Retrieved from