Introduction
With the gradual development of science and technology and the popularization of the use of the network, the search engine has become the most direct way for people to obtain accurate information in a huge amount of information in a hurry, it is only necessary to want to understand the content of the relevant information entered into the search engine, it will jump to the corresponding content of the information page for the user to read. But the apparently fair and impartial search engine also exists due to certain factors including (political management, stereotypes) and other factors caused by bias in favor of certain results rather than other results.
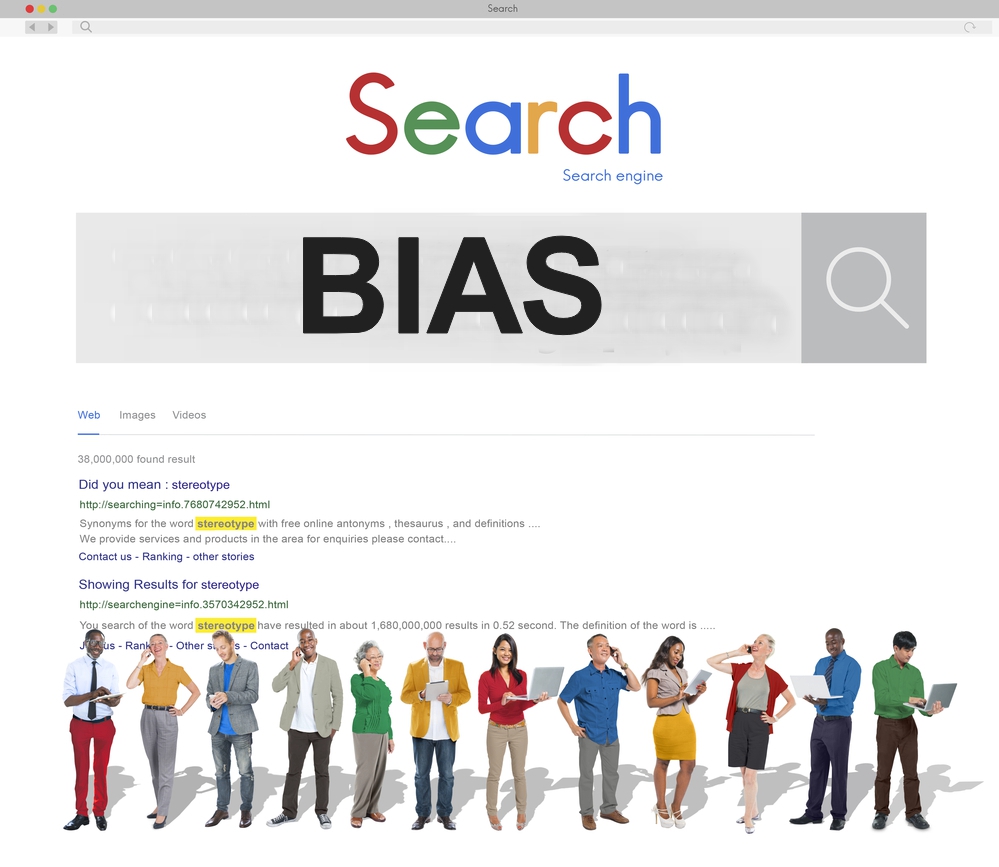
There are two difference aspect for harmful basis of the search engine.
Reinforce stereotypes: Biased search results reinforce stereotypes and lead to discrimination and bias.
Inequality in Access: Marginalized communities may struggle to find information that matters to them, exacerbating existing inequalities.
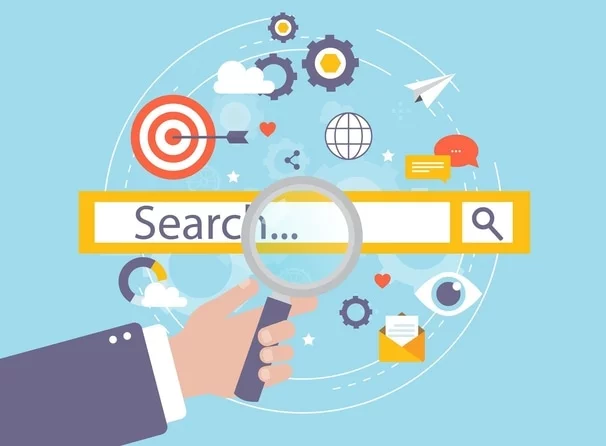
Reinforce stereotypes
The bias caused by search engines directly leads to deepening stereotypes. Including racial bias and gender bias.
Racial bias in search engines is an inevitable reality, and it is most prominently reflected in Google’s search engine. Here are two type-related examples:
For example, an MBA student named Bonnie Kamona reported that “a Google Images search for “unprofessional hair for work” brought up a set of images that almost exclusively depicted women of color. (Otterbacher,2016)
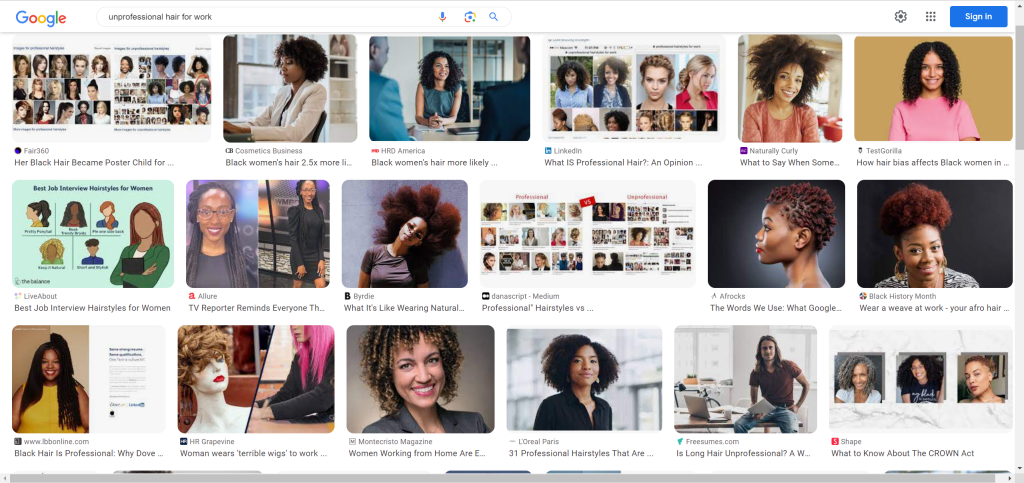
However, she searches for “professional hair” brought up images of white women. professional hair” brought up images of white women.”(Otterbacher,2016)
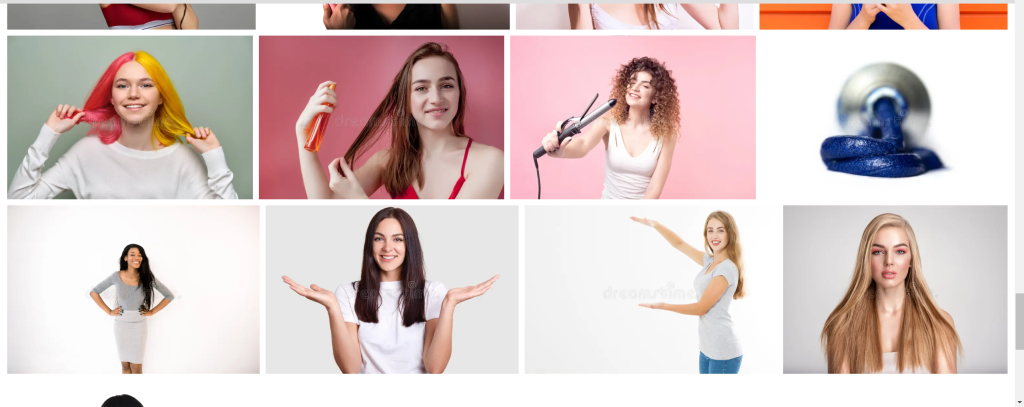
This difference illustrates the discrimination and bias that Google’s search engine has towards some women of color.
There are also other examples of the same type of discrimination.
such as “when twitter user Ali Kabir searched for “three black teenagers” on the search engine and was shown a large number of mugshots, which did not seem to match the content of the search. The headshots give off a disrespectful behavior.” (Otterbacher,2016)
Whereas, he searched for “three white teenagers” and got very different results, showing images of young people having fun. (Otterbacher, 2016)
Example video
What emerges from these differences is that racial discrimination in society and online continues to reinforce stereotypes about these specific groups of people. (Otterbacher, 2016)
Gender bias is very strong in places such as Central Asia, especially in terms of treatment and pay. It also includes politics and the distribution of household chores and care. It is not only in stereotypes, but also in the Internet age, when search engines are the first means of accessing information. (United Nations Europe and Central Asia, 2018)
In the words of Otterbacher (2017) and Chen et al. (2018), “the results displayed by search engines re-emerge or reinforce the development of gender inequality regulations and real-life examples of these employment and business decision-making roles.”
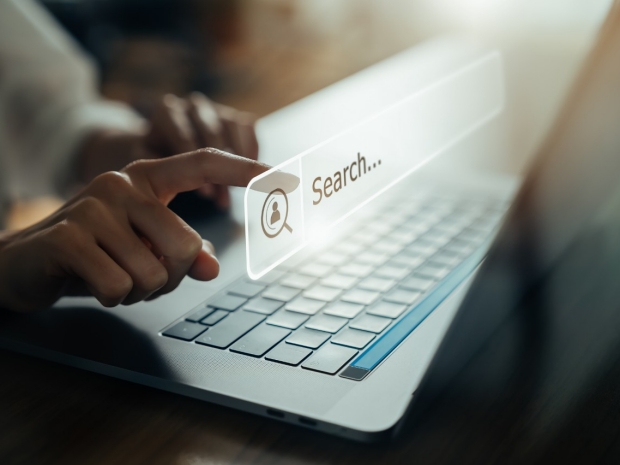
A recent study by a group of psychological researchers found that search engine results on the Internet, while ostensibly gender-neutral, produce a male-dominated content. This situation will continue to bring about a negative impression of gender equality, which will affect the employment opportunities and salary issues in the society. (NYU, 2022)
All of these types of racial as well as gender-based discrimination reinforce stereotypes of particular groups in modern society. For example, search engines associate the term “black community” with the term “chimpanzee”, as shown in the following image. This seems to be a very disrespectful and even insulting behavior towards specific groups.
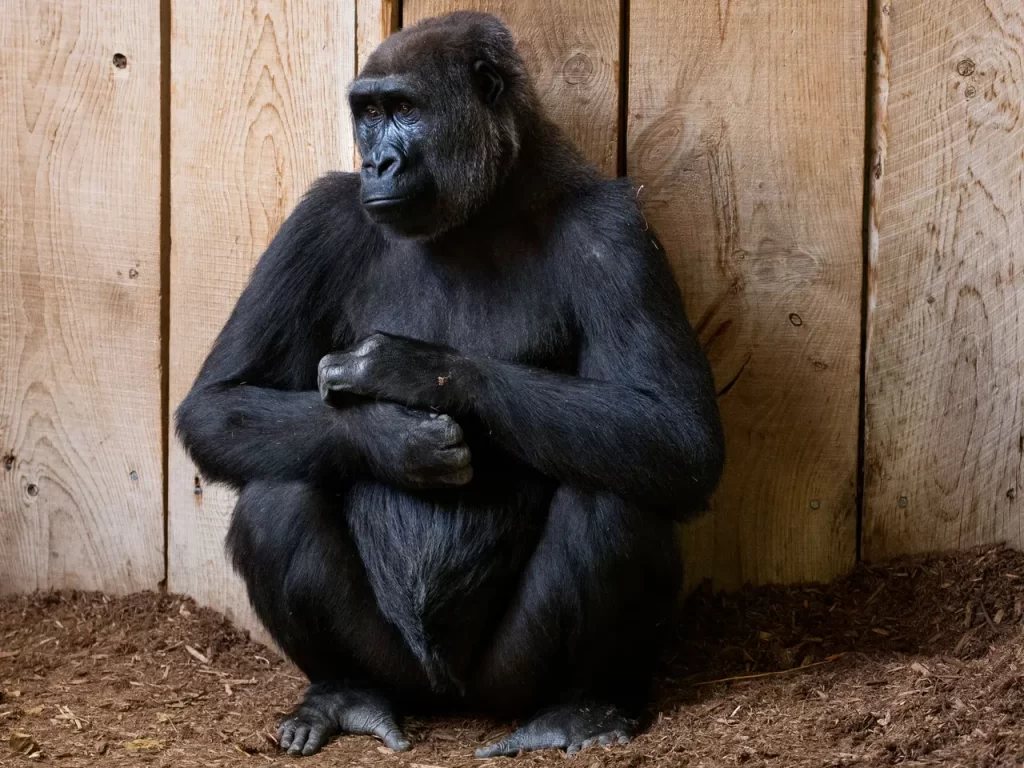
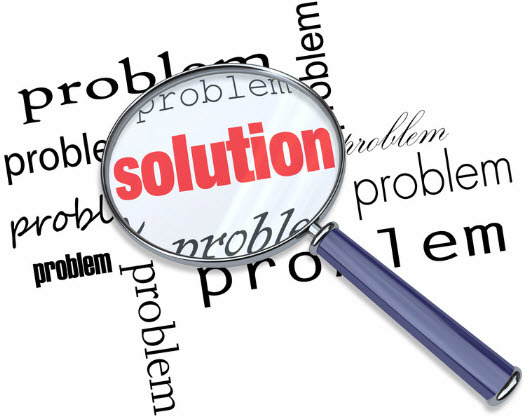
Solution
These biases are caused by the fact that gender bias and racial discrimination have been programmed into search algorithms. If we want to reduce discrimination and bias in search engines. Firstly, we need to get operators and stakeholders to work out ways to prevent users from being harmed by algorithmic bias. Secondly, we need to set up legislation and governmental bodies to regulate these algorithms to minimize the impact of bias and discrimination. (Lee et al, 2019)
Inequality of access to the information on search engine
Inequality of access to information for special groups on search engines is one of the more important issues now. It focuses on the difficulties faced by certain special groups in accessing information, services or resources through search engines. The main concern here is the inequality caused by the algorithmic bias of search platforms.
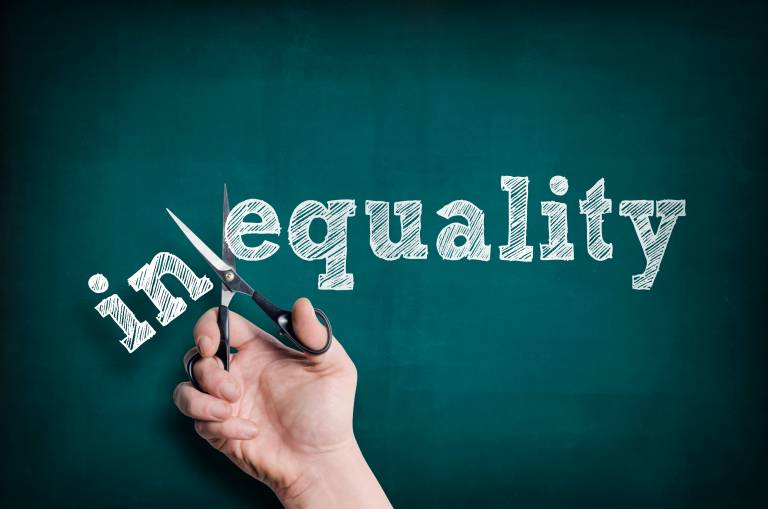
Two aspects contribute to inequality of access to information
- Cultural and Linguistic bias
- Relevance bias
Cultural and Linguistic bias
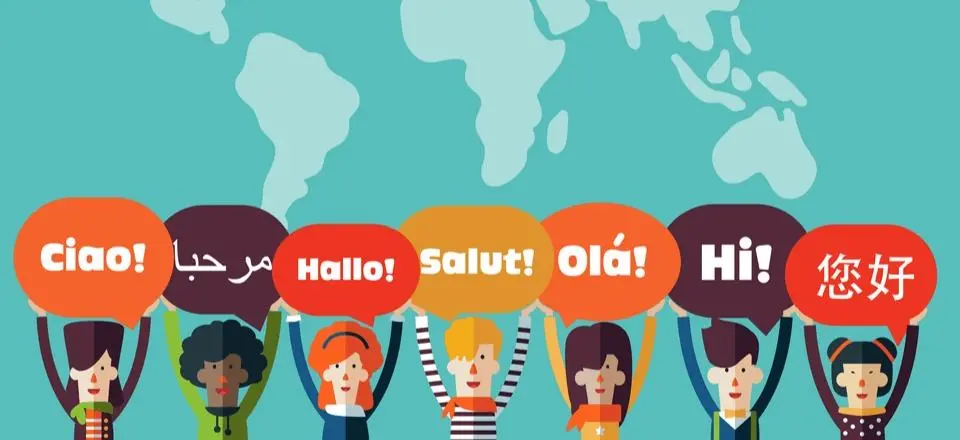
It is well known that the main language used in most search engines is English. If you use other languages, the results are very limited. Even though it is not possible to require everyone to be proficient in English, those who have English as a second language or are non-native speakers face difficulties in searching as compared to those who are native English speakers.
According to some of the non-native English speakers who participated in the study, “It is sometimes difficult to express oneself in English, especially when it comes to English terminology.” Most importantly, they said, “when searching for information, there is a high probability that the results will be biased due to the inability to enter key words or phrases in English.”(Chu et al, 2016)
This situation results in other non-native English speakers not being able to accurately access the information that they really need. As a result, they are unable to participate in research on esoteric topics and can even be excluded from discussions by native English speakers.
According to an Ethiopian-accented staff member of a global non-profit organization, he was often interrupted and excluded from meetings by colleagues who commented on his incomprehensible English because of his inability to express himself in English. (Ro, 2021)
Relevance bias
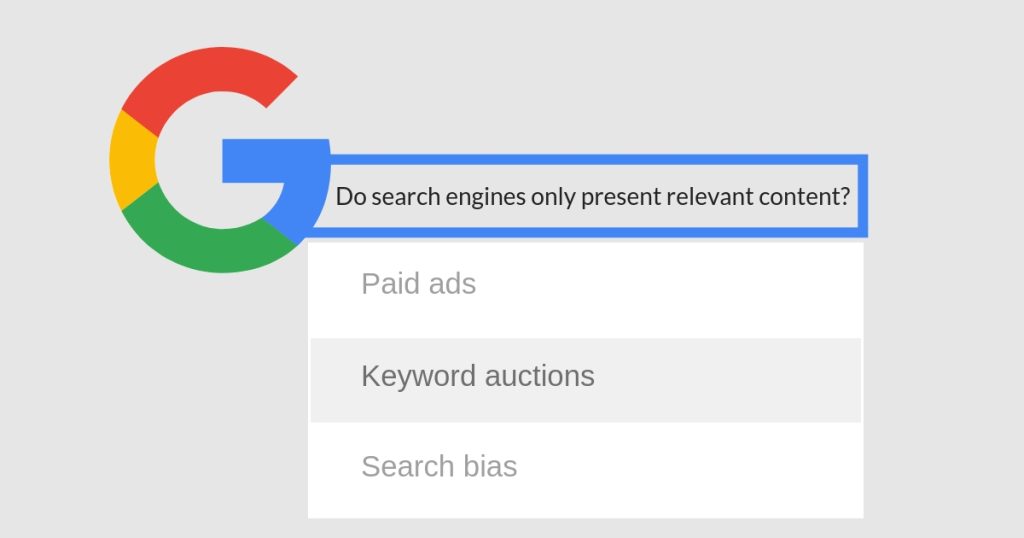
Another factor that affects the inequality of access on search engines is content relevance bias. This factor leads to the fact that search engines tend to present information that most people identify with and frequently browse, resulting in the amplification of mainstream information at the expense of specialized or niche information.
According to the example pointed out by Yilmza et al. (2015), when a user enters a query for certain information, such as “coffee health”. The benefits and the drawbacks of coffee should be shown so that people are fully aware of the pros and cons of coffee. However, this is not the case with the Google search engine, which presents only the benefits of coffee at the top of the results page, biasing the public’s perception and leading them to believe that the benefits of coffee outweigh the drawbacks or even only bring benefits. Ultimately, this makes it difficult for the public to access the full range of information and creates an unfair bias towards the content of the search.
This situation seems to be harmless, in fact, Google search engine this kind of mainstream information content amplification, reduce the dissemination of niche information in the region of the situation is subconsciously affecting the public’s thinking, resulting in the proliferation of prejudice.
At the same time, it also shows the problem of unfair access to information by search engines. This problem can be solved by diversifying searches and comparing results between search engines. Firstly, users can search for different aspects of topics and issues of interest, such as modifying keywords or searching for topics related to different communities and cultures to get comprehensive information. Secondly, users can also search for the same topics or keywords on different search engines such as Bing or Edge. Finally, the results they produce are compared to discover the differences.
Conclusion
Overall, it has explored the phenomenon of search engines leading to the reinforcement of stereotypical influences as well as the issue of algorithmic discrimination against non-native English speakers for cultural as well as linguistic differences and incomplete search engine results indirectly leading to the perpetuation of harmful biases in search engines. Online users and creators should take proactive measures and demand fairness and transparency in search engine algorithms to mitigate the perpetuation of bias or exacerbate it. Users should also be urged to question the results of search engines and not to believe everything they read.
Reference list
- Chen L., Ma R., Hannák A., Wilson C. (2018). “Investigating the Impact of Gender on Rank in Resume Search Engines,” in Proceedings Of the 2018 CHI Conference On Human Factors In Computing Systems. Editors Mandryk R., Hancock M. (Montreal QB, Canada: Australian National University Press; ), 1–14.
- Chu,P., Komlodi,A., Rózsa,G. (2016) Online search in english as a non-native language. https://asistdl.onlinelibrary.wiley.com/doi/full/10.1002/pra2.2015.145052010040
- Lee,T,N., Resnick,P., Barton,G., (2019). Algorithmic bias detection and mitigation: Best practices and policies to reduce consumer harms. https://www.brookings.edu/articles/algorithmic-bias-detection-and-mitigation-best-practices-and-policies-to-reduce-consumer-harms/
- NYU. (2022). Gender Bias in Search Algorithms Has Effect on Users, New Study Finds. https://www.nyu.edu/about/news-publications/news/2022/july/gender-bias-in-search-algorithms-has-effect-on-users–new-study-.html#:~:text=The%20results%20showed%20that%20the,tracks%20with%20societal%20gender%20inequality.
- Otterbacher, J., (2016). New Evidence Shows Search Engines Reinforce Social Stereotypes. https://hbr.org/2016/10/new-evidence-shows-search-engines-reinforce-social-stereotypes
- Otterbacher J., Bates J., Clough P. (2017). “Competent Men and Warm Women: Gender Stereotypes and Backlash in Image Search Results,” in Proceedings Of the 2017 Chi Conference On Human Factors In Computing Systems. Editors Mark G., Fussell S. (Denver, CO, United States:ACM New York; ), 6620–6631
- Shokouhi,M., White,R., Yilmaz,E., (2015). Anchoring and Adjustment in Relevance Estimation. https://dblp.org/rec/conf/sigir/ShokouhiWY15.html.
- UNDP, United Nations Europe and Central Asia (2018). United Nations Development Programme Focus Gender Equality. Available at: http://www.eurasia.undp.org/content/rbec/en/home/gender-equality.html.