Introduction
The convergence of Indigenous knowledge, stolen artwork, and the emerging field of artificial intelligence presents a complex and thought-provoking issue in contemporary society (Carlson & Richards, 2023). Indigenous cultures globally possess a wealth of knowledge, traditions, and creative expressions that have immense value and are deeply rooted in their cultural identity.However, the legacy of this phenomenon has been marred by a history of theft, appropriation, and exploitation. Furthermore, the growth of AI and machine learning technology has brought to the forefront concerns around cultural insensitivity, biased algorithms, and the potential harm inflicted on Indigenous communities (Kaissis et al., 2020). This paper will explore strategies for addressing these challenges, such as using Indigenous knowledge to mitigate art theft and promote culturally sensitive AI practices. These strategies include collaborating with Indigenous communities, obtaining legal ownership of traditional knowledge, collecting comprehensive data, creating artificial intelligence under Indigenous leadership, implementing repatriation projects, safeguarding digital material, mitigating prejudice, and offering culturally sensitive training. These techniques can bridge the divide between technology and Indigenous cultures while fostering principles of fairness, reverence, and ethical ingenuity. This essay will discuss these strategies to harness Indigenous knowledge to mitigate stolen art and culturally unsafe AI practices while providing counterarguments.
Harnessing Indigenous Knowledge
Establishing a strong basis of cultural respect and collaboration with Indigenous communities is a primary strategy in mitigating instances of art theft and the perpetration of culturally detrimental behaviors by artificial intelligence systems (Carlson & Richards, 2023). Further, recognizing the significance of Indigenous knowledge and art within their cultural contexts requires the utmost respect and consideration. To get a comprehensive understanding of Indigenous practices, beliefs, and worldviews, engaging in dialogue with esteemed elders, leaders, and experts in the respective subject is essential (Kaissis et al., 2020). Nevertheless, it is essential to establish legal frameworks that acknowledge and safeguard traditional knowledge to mitigate theft and appropriation of Indigenous knowledge. Additionally, these frameworks must have provisions granting them intellectual property rights about their knowledge and creative expressions to facilitate the autonomy and authority of Indigenous groups concerning the use and transmission of their cultural legacy (Zeadally et al., 2020). The implementation of domestic legislation and adherence to international agreements may significantly facilitate the attainment of this objective.
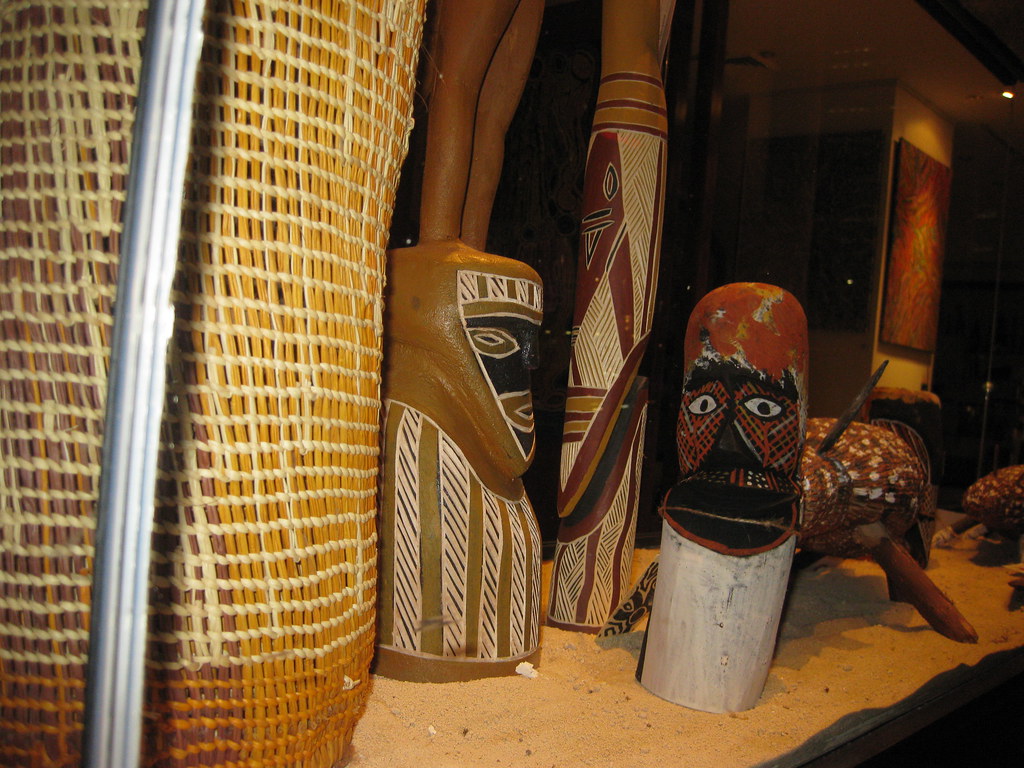
“INDIGENOUS ART” by RubyGoes is licensed under CC BY 2.0.
Moreover, the use of extensive data is often seen in the development of artificial intelligence systems (Carlson & Richards, 2023). Ensuring the inclusivity and accurate representation of Indigenous cultures inside databases is critical to mitigate prejudice and cultural insensitivity. To ensure the collection and effective management of data that authentically reflects the experiences and perspectives of Indigenous people, it is essential to establish a strong collaborative relationship with them (Adi et al., 2022). Similarly, cultural sensitivity should be included in the development of AI systems to ensure the accurate interpretation and processing of data. Besides, addressing the negative cultural implications of AI practices can be instrumental in empowering Indigenous communities to take the lead in AI development endeavors that include their knowledge and cultural heritage (Carlson & Richards, 2023). They can develop expertise in artificial intelligence by providing indigenous communities with access to resources, training, and opportunities. This approach will facilitate the incorporation of cultural values and needs into the development of the technology.
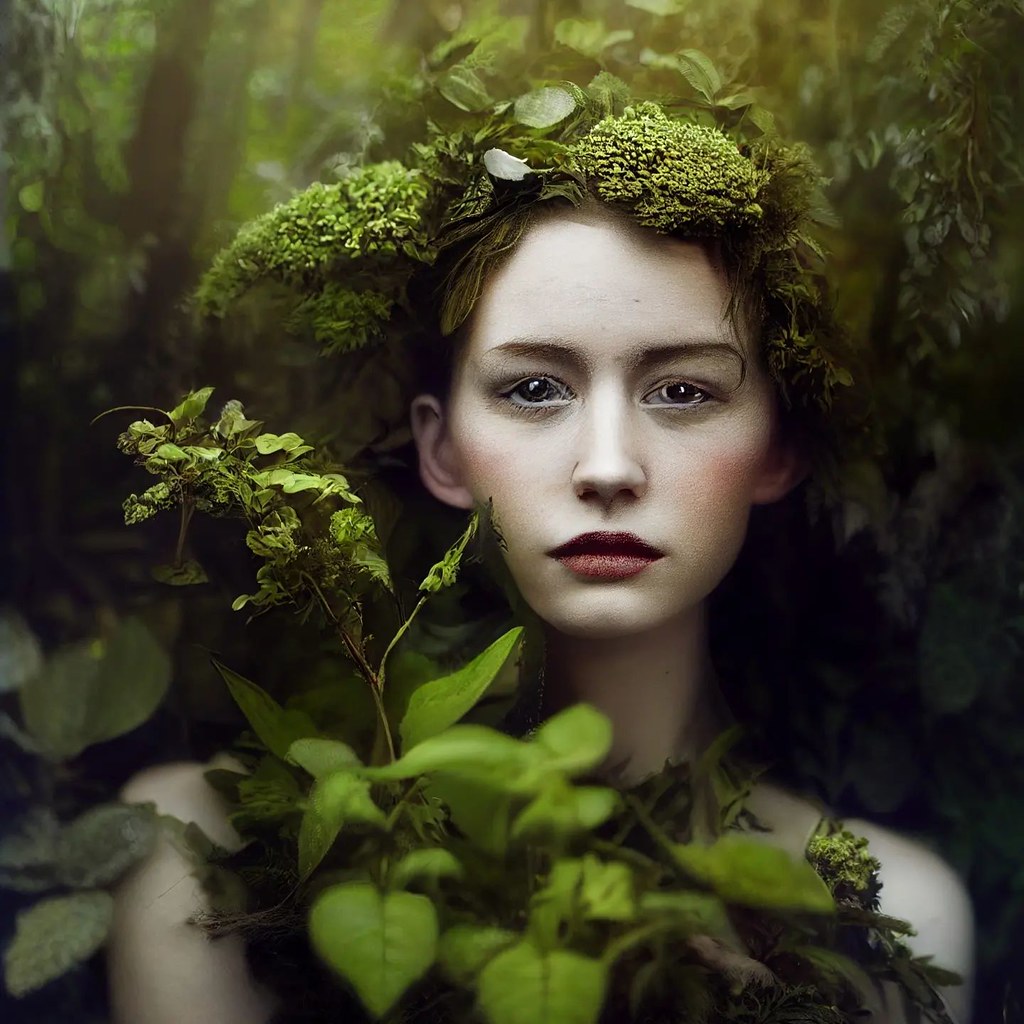
“spielt mit #textToImage #AI : #midJourney vs. #stableDiffusion Der verwendete Textpromt ist jedesmal gleich :’young and beautiful woman dressed in flowers and herbs, full body, lying, Green eyes, fine art, detailed, Venus, Annie Leibovitz style, National” by der sich den wolf tanzt is licensed under CC BY-SA 2.0.
Mitigating Stolen Art
The issue of theft and misappropriation has plagued Indigenous art for an extended period, with several instances of Indigenous artworks being held in private and museum collections without the consent of their particular communities (Carlson & Richards, 2023). Notably, there is a need for heightened endeavors to facilitate the repatriation of these unlawfully acquired art pieces to their legitimate proprietors. It is important to include Indigenous communities in this process to enable their participation in decision-making about preserving and managing their cultural assets (Zeadally et al., 2020). Additionally, digital reproductions and online exhibitions can be created to preserve and distribute Indigenous art while preserving the integrity of the original works. Indigenous artists use modern technologies to communicate their narratives, showcase their artistic creations, and educate a global audience about their cultural heritage (Kaissis et al., 2020). This approach maintains the integrity of the artwork while also enabling broad accessibility.
Culturally Safe AI Practices
Artificial intelligence systems tend to exhibit biases perpetuating cultural insensitivity and promoting preconceptions (Dwivedi et al., 2021). Comprehensive examination and evaluation of AI algorithms are necessary to mitigate biases by identifying and rectifying any biased outcomes. To ensure fairness and promote cultural awareness, this method must include perspectives from many groups, including experts from Indigenous communities. Furthermore, AI developers and practitioners need to undergo cultural sensitivity training to understand the intricacies and intricacies of Indigenous cultures (Carlson & Richards, 2023). The use of this training may enable individuals to guide the development of AI systems in alignment with Indigenous values and perspectives, hence mitigating the risk of unintentional cultural insensitivity.
Counterargument
The key counterargument to harnessing Indigenous knowledge is that challenges stemming from cultural differences and logistical complexities might pose significant barriers to establishing such cooperation. There may be a question about the preparedness of Indigenous communities to collaborate with digital corporations and educational institutions that have historically exploited their knowledge and skills (Goldstein et al., 2023). In light of this counterargument, it is imperative to emphasize the significance of cultivating trustworthy connections and fostering genuine partnerships that maintain the principles of Indigenous sovereignty and knowledge ownership. A viewpoint suggests that the provision of intellectual property rights to Indigenous groups might hinder their capacity for innovation and restrict the dissemination of knowledge (Pagano et al., 2023). Further, there is a contention among some individuals that implementing these protective measures might potentially discourage the development of artificial intelligence applications designed to uphold and bolster Indigenous customs and practices (Kaissis et al., 2020). Therefore, it is essential to prioritize the development of responsible innovation that demonstrates due regard for cultural boundaries and concurrently protects traditional knowledge.
Moreover, some critics have argued that collecting data from Indigenous communities might potentially infringe upon their privacy and be considered intrusive (Zeadally et al., 2020). One may also question the feasibility of gathering comprehensive data from diverse Indigenous groups. Hence, it is imperative to prioritize informed consent, data anonymization, and respectful data-sharing agreements to tackle privacy concerns and ensure ethical data collection. Similarly, critics have argued that Indigenous communities may lack the requisite technical expertise to manage AI development programs effectively (Dwivedi et al., 2021). The potential skepticism over the feasibility of this method may arise due to the significant digital divide prevalent among Indigenous communities.
Another counterargument is that several artworks possess intricate provenances and histories, making identifying rightful ownership challenging, hence asserting that repatriation efforts are too simplistic (Dwivedi et al., 2021). Furthermore, one can contemplate the feasibility of repatriating all pilfered artwork. Consequently, to formulate equitable resolutions, it is imperative to acknowledge the intricacy of the matter and engage in cooperative efforts with Indigenous communities. According to some perspectives, digital reproductions are deemed insufficient to capture original artworks’ cultural significance and authenticity. Individuals may also express concerns about the potential exploitation of digital copies (Pagano et al., 2023). In light of this counterargument, it is imperative to emphasize that digital initiatives are intended to augment conventional artistic mediums and serve as instruments for cultural conservation and pedagogy rather than wholly supplanting them.
Conclusion
Developing culturally appropriate artificial intelligence approaches necessitates the dual consideration of preserving Indigenous art and incorporating Indigenous knowledge. The promotion of a more equitable and culturally sensitive AI landscape can be achieved by implementing various strategies. These strategies encompass cultural respect, collaboration, protection of traditional knowledge, inclusive data practices, Indigenous-led AI development, repatriation efforts, digital reproductions, bias mitigation, and cultural sensitivity training. However, it is essential to recognize and respond to counterarguments by careful consideration, collaboration, and a commitment to ethical and courteous behavior. Ultimately, Indigenous knowledge offers a means to promote diversity, inclusivity, and ethical progress in artificial intelligence and machine learning while concurrently mitigating instances of art theft and cultural insensitivity.
References
Adi, E., Baig, Z., & Zeadally, S. (2022). Artificial Intelligence for Cybersecurity: Offensive Tactics, Mitigation Techniques and Future Directions. Applied Cybersecurity & Internet Governance, 1(1), 1-23. https://acigjournal.com/resources/html/article/details?id=232841&language=en
Carlson, B., & Richards, B. (2023, Sep. 8). Indigenous knowledges informing ‘machine learning’ could prevent stolen art and other culturally unsafe AI practices. The Conversation. https://theconversation.com/indigenous-knowledges-informing-machine-learning-could-prevent-stolen-art-and-other-culturally-unsafe-ai-practices-210625
Dwivedi, Y. K., Hughes, L., Ismagilova, E., Aarts, G., Coombs, C., Crick, T., … & Williams, M. D. (2021). Artificial Intelligence (AI): Multidisciplinary perspectives on emerging challenges, opportunities, and agenda for research, practice and policy. International Journal of Information Management, 57, 101994. https://doi.org/10.1016/j.ijinfomgt.2019.08.002
Goldstein, J. A., Sastry, G., Musser, M., DiResta, R., Gentzel, M., & Sedova, K. (2023). Generative language models and automated influence operations: Emerging threats and potential mitigations. arXiv preprint arXiv:2301.04246. https://doi.org/10.48550/arXiv.2301.04246
Kaissis, G. A., Makowski, M. R., Rückert, D., & Braren, R. F. (2020). Secure, privacy-preserving and federated machine learning in medical imaging. Nature Machine Intelligence, 2(6), 305-311. https://www.nature.com/articles/s42256-020-0186-1
Pagano, T. P., Loureiro, R. B., Lisboa, F. V., Peixoto, R. M., Guimarães, G. A., Cruz, G. O., … & Nascimento, E. G. (2023). Bias and unfairness in machine learning models: a systematic review on datasets, tools, fairness metrics, and identification and mitigation methods. Big data and cognitive computing, 7(1), 15. https://doi.org/10.3390/bdcc7010015 Zeadally, S., Adi, E., Baig, Z., & Khan, I. A. (2020). Harnessing artificial intelligence capabilities to improve cybersecurity. Ieee Access, 8, 23817-23837. https://ieeexplore.ieee.org/abstract/document/8963730