Topics:Algorithms , Social Media
Posted by: Jiaqi Wang TUT11
Introduce
Background information
In today’s digital age, search engines and social media platforms have become indispensable tools for information retrieval and social interaction. They are gateways to a world of knowledge and connectivity, influencing the way we think, feel and participate in society. However, a growing body of research and reporting in recent years has revealed harmful biases in how these platforms handle information and content recommendations. From gender to race to political affiliation, these biases not only limit users’ access to diverse perspectives, but they can also exacerbate social inequality and discrimination. For example, as shown in Figure 1, there is gender inequality in searches in search engines in different countries. In some countries, such as Turkey, the proportion of male images appearing in search results is much higher than in other countries (Vlasceanu, M. and Amodio, 2022). Therefore, this essay attempts to explore the key issues and countermeasures for harmful biases in search engines and social media platforms.
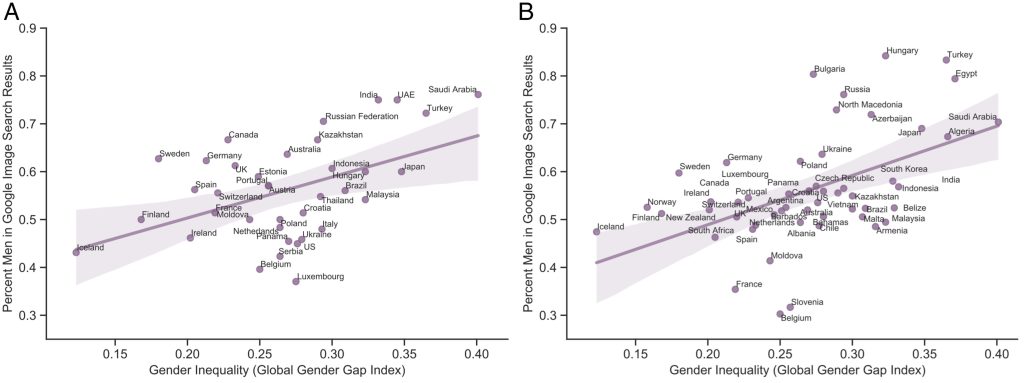
Figure 1: Gender inequality in search engines (Vlasceanu, M. and Amodio, 2022)
Search engine bias
“Harmful bias in search engines” refers to search engines’ systematic and unfair favoritism or discrimination against certain groups, ideas, or content (DeVos et al., 2022). The underlying basis of search engines is algorithms, so the bias of algorithms is an important reason for the appearance of Harmful Biases in search engines. Algorithmic bias refers to systematic and repeatable errors in computer systems that produce unfair outcomes, such as favoring any one group of users over others. In the context of search engines, this bias can manifest in the ranking and display of search results, thereby affecting the information users are most likely to interact with (Baeza-Yates, 2018). For example, a search query for “best scientists” may disproportionately show male scientists due to historical gender biases embedded in the algorithm, as shown in Figure 2. Another example is Typing “Asian girls” into Google. This search query brings up pornographic and highly sexualized results, highlighting the issue of content that pops up on the first page of Google as shown in Figure 3.
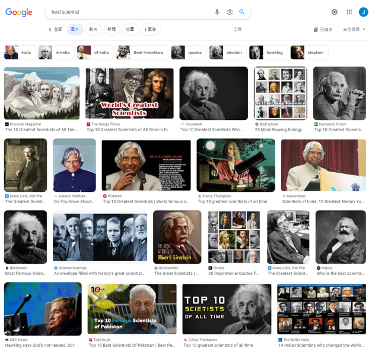
Figure 2: Best scientist search results
Source: google search results
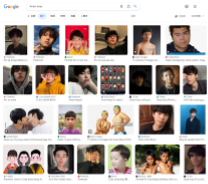
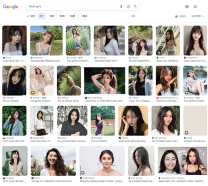
Figure 3: Search results for Asian boys and Asian girls
Source: google search results
Additionally, a notable example of search engine bias is Google’s autocomplete feature, which has been criticized for perpetuating stereotypes. For example, typing “Why do Women” may yield negative or disparaging suggestions, as shown in Figure 4.
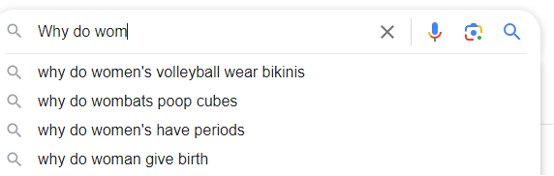
Figure 4: Google search results association
Source: google search
Search engine bias is a complex issue rooted in multiple factors interacting in subtle ways. One of the main culprits is data bias, where algorithms inherit biases present in the data they were trained on. Designer bias exacerbates this situation, as human programmers may unintentionally encode their own opinions and biases into the algorithms they create (Shen et al., 2021). User behavior also plays a role; search engines optimize their algorithms based on user clicks, which may reflect and amplify social biases. Commercial motives may further skew results, as search engines may prioritize paid content or advertisers, thereby introducing another layer of bias (Mays, 2014). Personalized search, which tailors results based on a user’s search history and personal information, can reinforce existing biases by creating “filter bubbles” that isolate users’ different perspectives. Social and cultural factors such as gender, race, and location also influence search results. These multi-layered biases not only affect the information we receive, but also exacerbate social inequalities and cultural biases.
Search engine bias can have profound social and cultural consequences. By perpetuating stereotypes and limiting exposure to diverse perspectives, these platforms may exacerbate social inequalities (Mays, 2014). For example, if search engines primarily show high-paying job listings to men, this could perpetuate gender income inequality. Likewise, by reinforcing existing beliefs through personalized search results, search engines may contribute to ideological polarization, making social consensus more difficult to achieve.
Bias on social media platforms
Recommendation algorithms in social media platforms curate and present content based on users’ past behavior, preferences and network connections. While these algorithms are designed to increase user engagement, they can inadvertently exacerbate existing biases(Hargittai, 2020). For example, if a user frequently interacts with politically conservative content, the algorithm may recommend more of the same content, thereby reinforcing the user’s existing beliefs and biases. Facebook’s news feed and Twitter’s trending topics are prime examples of how bias can manifest in social media recommendations. Research shows that Facebook’s algorithms can create “echo chambers,” a phenomenon in which individuals or groups communicate with each other in a closed information environment that reinforces their existing opinions and beliefs. This phenomenon is particularly evident on social media platforms, where algorithms often personalize information flows based on users’ behavior and preferences, resulting in users being exposed to more information that aligns with their own views and only being exposed to information similar to their own. the opinion of. Twitter’s trending topics have also been criticized for sometimes promoting misleading or biased narratives, especially during political events or social movements. Figure 5 shows Conceptualisation of echo chambers through confirmation bias on social media.
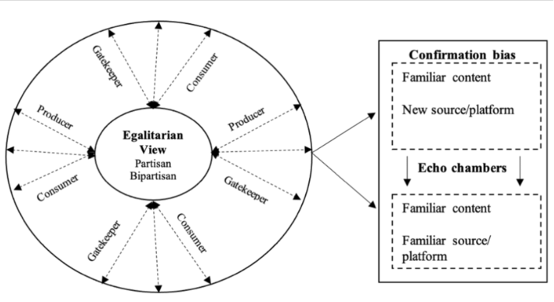
Figure 5: Conceptualization of echo chambers through confirmation bias on social media(Modgil et al., 2021)
The effects of social media echo chambers are manifold and concerning. First, they lead to a polarization of opinions. By continually exposing individuals to views similar to their own, echo chambers reinforce existing beliefs, making them more extreme over time. Second, they facilitate the spread of misinformation and prejudice. In a closed loop of like-minded individuals, false information can spread without questioning and gain inappropriate credibility (Terren & Borge-Bravo, 2021). Ultimately, these factors combine to cause social fragmentation. The deepening of ideological differences and the spread of false information may weaken social cohesion, leading to a more divided and disharmonious society. The consequences of echo chambers therefore extend beyond individual beliefs and affect broader social structures (Cinelli et al., 2021).
Solutions and Challenges
Several solutions have been proposed to address bias in search engines and social media platforms. One approach is algorithmic transparency, requiring companies to disclose how their algorithms work. Another solution is to use “fairness-aware” algorithms designed to minimize or eliminate bias (Le Quy et al., 2022). Regulatory oversight is also under consideration, with government agencies monitoring and auditing algorithms for biased behavior (Shneiderman, 2016). Additionally, user education can empower individuals to become more discerning consumers of digital content, helping them recognize and overcome biases.
Despite these proposed solutions, addressing bias in digital platforms is a complex and multifaceted issue. First, algorithms are often proprietary, making transparency a challenging requirement for companies that treat this information as trade secrets. Second, while fairness-aware algorithms sound promising, defining “fairness” itself is a controversial issue, influenced by cultural and social variables. Additionally, regulatory oversight raises concerns about free speech and innovation. Finally, user education is a long-term solution that requires systemic changes to digital literacy programs and is therefore difficult to implement quickly.
Conclusion
In summary, search engines and social media platforms, while valuable tools for information and social interaction, are also riddled with harmful biases that perpetuate social inequality and ideological polarization. These biases are deeply embedded in algorithms, data, and user behavior, making them difficult to address. Solutions such as algorithmic transparency, fairness-aware algorithms, and regulatory oversight offer promising avenues, but also bring their own set of challenges. Ultimately, a multi-pronged approach that combines technological innovation, regulatory oversight and user education is critical to mitigating the adverse effects of these biases and fostering a fairer digital environment.
Reference
Baeza-Yates, R., 2018. Bias on the web. Communications of the ACM, 61(6), pp.54-61.https://doi.org/10.1145/3209581
Cinelli, M., De Francisci Morales, G., Galeazzi, A., Quattrociocchi, W. and Starnini, M., 2021. The echo chamber effect on social media. Proceedings of the National Academy of Sciences, 118(9), p.e2023301118. https://doi.org/10.1073/pnas.2023301118
DeVos, A., Dhabalia, A., Shen, H., Holstein, K. and Eslami, M., 2022, April. Toward User-Driven Algorithm Auditing: Investigating users’ strategies for uncovering harmful algorithmic behavior. In Proceedings of the 2022 CHI Conference on Human Factors in Computing Systems (pp. 1-19). https://doi.org/10.1145/3491102.3517441
Hargittai, E., 2020. Potential biases in big data: Omitted voices on social media. Social Science Computer Review, 38(1), pp.10-24. https://doi.org/10.1177/0894439318788322
Le Quy, T., Roy, A., Iosifidis, V., Zhang, W. and Ntoutsi, E., 2022. A survey on datasets for fairness‐aware machine learning. Wiley Interdisciplinary Reviews: Data Mining and Knowledge Discovery, 12(3), p.e1452. https://doi.org/10.1002/widm.1452
Mays, L., 2014. The Consequences of Search Bias: How Application of the Essential Facilities Doctrine Remedies Google’s Unrestricted Monopoly on Search in the United States and Europe. Geo. Wash. L. Rev., 83, p.721.
Modgil, S., Singh, R.K., Gupta, S. and Dennehy, D., 2021. A confirmation bias view on social media induced polarisation during Covid-19. Information Systems Frontiers, pp.1-25.
Shen, H., DeVos, A., Eslami, M. and Holstein, K., 2021. Everyday algorithm auditing: Understanding the power of everyday users in surfacing harmful algorithmic behaviors. Proceedings of the ACM on Human-Computer Interaction, 5(CSCW2), pp.1-29. https://doi.org/10.1145/3479577
Shneiderman, B., 2016. The dangers of faulty, biased, or malicious algorithms requires independent oversight. Proceedings of the National Academy of Sciences, 113(48), pp.13538-13540. https://doi.org/10.1073/pnas.1618211113
Terren, L., & Borge-Bravo, R. (2021). Echo Chambers on Social Media: A Systematic Review of the Literature. Review of Communication Research, 9, 99–118. Retrieved from https://rcommunicationr.org/index.php/rcr/article/view/94
Vlasceanu, M. and Amodio, D.M., 2022. Propagation of societal gender inequality by internet search algorithms. Proceedings of the National Academy of Sciences, 119(29),